The most used is, by far, the dermatoscopy, or epiluminescence microscopy, which consists on the exam of the skin through the utilization of an optical system and a light source designed to reduce the irregular refraction and the light reflection in its surface. Thus, it is possible to visualize in depth structures, forms and colors which are not accessible to the visual inspection. The dermatoscopy has been demonstrated especially useful to define the benignity or malignancy of the pigmented lesions. The device used is called dermatoscope which, as it can be seen in Fig. 1, connected to a computer captures the images, existing two types of taken images, those of the dermatoscopy with contact illumination type and non-polarized light, using a liquid in the interphase, and those of the dermatoscopy with cross-polarized light, for which it is needed the dermatoscope to be equipped with polarized light lenses, with a cross polarization filter [3]. Although there are many differences, most of the structures and observed colors with contact dermatoscopy and polarized light dermatoscopy are equivalent.
This method presents better results than the “naked eye” analysis. In expert hands a sensitivity of 89 % is achieved (against the 70–85 % obtained through the “naked eye”) [4]. This is an indicative of the robustness of this technique and means that most melanomas can be diagnosed, although it should always be taken into account that there is a percentage of 11 % of the cases in which it is not applicable. This system also allows the reproducibility in the diagnosis, enabling second opinions and allowing the use of image processing technologies.
There exist new promising techniques which are alternative to dermatoscopy [5–8], notwithstanding the easiness of image acquisition, its good results and its utilization level between medical experts ensures its utilization over a long period of time. In fact, dermoscopy has been recognized as the “gold standard” in the screening phase [8].
In the diagnosis of the melanoma over dermoscopic images the most used method is the 2-stage method. As it can be seen in Fig. 2, this method consists on, in a first stage, the dermatologist has to discern whether the lesion is melanocytic or not, with a series of criteria and, in a second stage, if so, to use a diagnostic method to determine the level of malignancy, with the aim of deciding if the skin lesion should be removed [3]. The 4 main methods used by the dermatologists are: “Pattern Analysis” [9, “ABCD Rule” [10], “Menzies Method” [11] and “7-Point Checklist” [12]. It is, in all of them, about to detect and to characterize a series of indicators present in the skin lesion image, determining the diagnosis in line with pre-established criteria. Notwithstanding, it should be noted that its objectivation is difficult, since, in many cases, the detection and characterization of these indicators are highly biased by the subjectivity of the dermatologists.
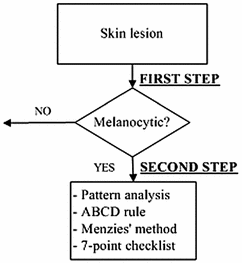
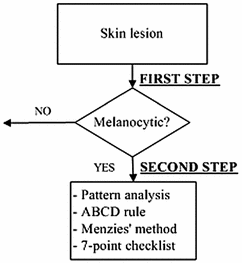
Fig. 2
Two steps procedure
It is clear that the automation of this process through computer aided diagnostic software is of great importance, since, on the one hand, it can accelerate the medical task, and on the other hand to provide a qualitative and objective evaluation of the skin lesion, decreasing the inter-observer and intra-observer variability which can be found in the diagnoses made by human experts.
Facing to the automation of the diagnosis, there exist a great number of algorithms which address different parts of this process, like certain processing or segmentation tasks, or the detection and characterization of indicators. However, there exist few software systems that address the automation of the whole process, and even less commercial tools which offer a certain degree of robustness in the diagnosis.
In this context, our research team has designed and developed a complete family of digital image processing algorithms which have been integrated in an automated system in support of the detection of melanoma, carrying out the help in the diagnosis over the quantitative base of the medical algorithm “ABCD rule”, also supporting the decision in the “Pattern Analysis”.
The results that are being obtained are very promising in the different stages of the diagnostic system: preprocessing, segmentation, detection, characterization of indicators and diagnosis.
Currently, this system is in piloting stage, and the aim is that the system can be applied in different areas. For instance, it can be used as a guide to medical professionals which are not experts in dermatology. The case of primary care physicians, or even if it is an expert dermatologist the task will be make it easier, either because of being great the number of moles to analyze or, simply, to give a second opinion. Another possible application is the execution of the system in massive analysis processes, over repositories of dermoscopic images, with the purpose of searching possible malign lesions. Or even in screening systems directed to the population in order to detect melanomas in early stages, over telemedicine platforms or from another type
In fact, the system has been designed in such a way that it is multiplatform, likely to be accessed by the doctor, apart from the desktop application, via web or mobile, to a server system centralized to the diagnoses. Furthermore, the system can be accessed by a normal user, via web or mobile, for screening objectives withstanding for this the analysis of macroscopic images: in fact, the system has a determined vocation towards the teledermatology, which is increasingly used.
This chapter has been structured as follows: in section “State of the Art”, a detailed review of the state of the art in the automated detection of melanoma is made, in section “System Design” the design of the proposed system is explained, in section “Results” the results of the system are presented, and, finally, in section “Conclusions and Future Work” the conclusions and the future work are showed.
State of the Art
In this section the state of the art in the automated detection of melanoma is presented. First of all, the aforementioned medical diagnostic methods are commented briefly, deepening in the “ABCD rule” and the “Pattern Analysis”. Secondly, the state of the art techniques for the automated detection of melanoma in dermoscopic images are explained in detail. Finally, a review of some of the most relevant commercial tools which currently exist for the early detection of melanoma is carried out.
Medical Methods in Dermoscopy: “Pattern Analysis” and “ABCD Rule”
As stated previously, in the diagnosis of melanoma over dermoscopic images the most used method is the 2 stage method, being these the most important 4 diagnostic methods used: “Pattern analysis”, “ABCD Rule”, “Menzies Method” and “7-Point Checklist”. It is about, in all of them, to detect and characterize a series of indicators, present in the image of the skin lesion, determining the diagnosis according to some established criteria.
The “Pattern Analysis” is the method by which the best results are obtained, since it allows a more complete approach of the pigmented lesions although, conversely, it is the one which requires more experience [3]. The other 3 methods are named medical algorithms, since in all of them it is about to detect and characterize quantitatively a series of indicators, present in the skin-lesion image, and thus assign them numerical scores, being the final value obtained the malignancy measurement result of the algorithm, determining the diagnosis according to pre-established value ranges. Obviously, owing to manage with characterizations and numerical punctuations, when approaching an automation of the diagnosis, the most appropriate is to implement a medical algorithm.
Pattern Analysis
It consists of finding the existing patterns in the lesion and taking a diagnostic decision. These patterns are visual structures with forms, colors and characteristic textures, as it can be seen in Fig. 3. It allows the recognition and valuation of a series of parameters and patterns which allow the dermoscopic distinction between a benign and a malignant lesion [9].
ABCD Rule
“ABCD Rule” is a medical algorithm that intends to facilitate diagnosis of melanoma to those observers less experienced in the technique. It is based on the valuation of 4 criteria [10], as can be seen in the Table 1:
Table 1
Criteria and punctuation of “ABCD rule”
Dermoscopic criteria | Score | Weighting/correction factor |
---|---|---|
Asymmetry | 0 to 2 | X 1, 3 |
Border | 0 to 8 | X 0, 1 |
Color | 1 to 6 | X 0, 5 |
Dermoscopic/dermoscopic structures | 1 to 5 | X 0, 5 |
Asymmetry: The pigmented lesion is divided in two perpendicular axes, in such a way that we achieve as much symmetry as possible, and the asymmetry is evaluated with respect to the shape, the color and the structure in both sides of each axis. A punctuation of 0 is granted if there is no asymmetry in any axis, of 1 if there exists asymmetry in one axis and of 2 if it presents it in both axes, in such a way that the lesion can have a score of 0–2.
Border: The lesion is divided in eight segments and it is scored with 1 each portion which might present an abrupt completion of the border. In such a way that the minimum score that it can be obtained is 0 and the maximum is 8.
Colors: It evaluates the presence of six colors (white, light brown, dark brown, blue-gray, red and black). Consequently, the maximal score will be 6 and the minimum 1. The white color will only be scored if it is lighter than the adjacent skin; that is, when it belongs to white areas of regression.
Dermoscopic Structures:
In the clinical ABCD, applicable to macroscopic images, the “D” is corresponding to the Diameter and it is considered a symptom of malignancy that it drops from 6 mm.
Here, we are describing the dermoscopic ABCD, in which the “D” is corresponding to dermoscopic structures, a similar concept to the “pattern” which it was considered in the “Pattern Analysis”. It is considered five structures, each of them scored with 1. Consequently the maximum score will be of 5 and the minimum of 1. The dermoscopic patterns are:
Subsequently each value is multiplied by each weighting factor, the partial scores are added, and the total dermoscopic score (TDS) is obtained.
Pigmented network (reticular): either if it is typical of atypical.
Homogeneous and unstructured areas: should cover more than 10 % of the lesion.
Points: should be more than two.
Globule: should be at least two.
Linear ramifications/pseudopods: should be more than two.
Once calculated the TDS, as can be seen in the Table 2, the diagnosis is determined, with the following ranges:
TDS
4.75: BENIGN

4.8
= TDS
= 5.45: SUSPICIOUS


5.45
TDS: MALIGNANT

Table 2
Diagnosis with “ABCD rule”
TDS | ||
---|---|---|
![]() | 4.8–5.45 | Techniques for the Automated Detection of Melanoma in Dermoscopic ImagesHere a state of the art of the techniques for the automated detection of melanoma in dermoscopic images used in the most relevant works is presented. To do so, first of all, the complete life cycle of an automated system in support of the detection of melanoma is going to be undertaken, and secondly, the techniques used in some of the most relevant works will be commented. Life Cycle of an Automated System for the Detection of MelanomaThe complete life cycle of an automated system for the detection of melanoma, using the dermoscopic technique, as can be seen in Fig. 4, consists on the following stages: ![]() Fig. 4 Tasks of the life cycle of an automated system for the detection of melanoma 1. Image acquisition: The acquisition of the dermoscopic image is carried out. 2. Image preprocessing: The preprocessing of the image is carried out. First of all, the problems of the improvement in the image quality are covered, with the aim of these representing as closely as possible the original one. Secondly, and it is here where the automated process of a software tool really begins, the problem in the detection and treatment of the “noise” is covered, which used to appear in this kind of images, such as hair, bubbles, flashes, shadows, ink marks in the skin, electronic marks (usually digital identifiers or copyright information), black frames and devices and rulers to measure. 3. Skin lesion segmentation: The skin lesion segmentation is carried out. Habitually, it is undertaken in an automated way. Notwithstanding, there exist semi-automated systems in which the expert is allowed to intervene in this stage, introducing information for the segmentation improvement. 4. Detection and characterization of indicators: The automated detection and characterization of the chosen indicators to undertake the diagnosis. 5. Diagnosis: On the basis of the medical algorithm, the quantitative calculation of the degree of malignancy is made to determine the diagnosis according to the ranges of the pre-established values. Techniques Used in Relevant WorksHere the techniques used in the 2–5 of the life cycle of a system of automated detection of melanoma will be explained. Image PreprocessingHere the problem in the detection and the treatment of the “noise” which sometimes appear in these type of images, detecting the devices existing in the image, and substituting the existing pixels in an appropriate manner. Due to its inevitability in the acquisition of images, the most relevant problem is, by far, the detection and treatment of hair, and in fact, it is the one which is treated more in the medical literature. The majority of works are based on the DullRazor ![]() In [18], Kiani et al. present a method for hair removal, better and more efficient than DullRazor ![]() In [19], Abbas et al. present a novel algorithm for detecting and repairing of hair-occluded information from dermoscopy images, which has a capability to preserve the skin lesion features such as color and texture. For the hair detection the method uses Gaussian and morphological filters, and for the restoration it uses a fast marching inpainting method. In [20], Abbas et al. undertake a comparative study between the previously presented method and the three main method of the state of art: linear interpolation, inpainting by nonlinear partial differential equation (PDE) and inpainting by exemplar-based repairing techniques. Lesion SegmentationFor the segmentation of the skin lesions within the dermoscopic lesion numerous methods have been proposed, corresponding to different approaches which the segmentation can be addressed with. In [21], Celebi et al. analyze the state of the art and the issue existing in the segmentation of the skin lesions in dermoscopic images. In this work the different segmentation methods are classified roughly in the following categories: 1. Histogram thresholding: obtaining an histogram threshold to make the segmentation; 2. Clustering: obtaining homogenous regions using color clustering algorithms; 3. Edge-based: using edge operators; 4. Region-based: obtaining homogenous regions using region merging, region splitting, or both; 5. Morphological: using the watershed transform; 6. Model-based: modeling of images as random fields; 7. Active contours (snakes and their variants): using active contours techniques; 8. Soft computing: using soft-computing techniques. In [22], Delgado et al. carry out the segmentation, using the IHP method, a method based on the accomplishment of a linear transformation over another color space in which the segmentation is made using techniques through histogram thresholding. With an idea similar to the previous one, in [23], Garnavi et al. undertake the projection of the dermoscopic image to different color channels, making subsequently the segmentation in two regions, through histogram thresholding, using the Otsu method, with the aim of obtaining the optimal projection. In [24], Celebi et al. propose a new method based on the fusion of some thresholding methods, using a Markov random field (MRF) framework, and subsequently this method is compared with nine methods of the state of art. In [25], Schmid et al. carry out the segmentation on the basis of the fuzzy c-means (FCM). In [26], Cucchiara et al. also use that method, paying the attention in issues of color, as well as topological ones, guiding the recursion of the algorithm by evaluation of adjacency and mutual inclusion properties of extracted regions. In [27], Zhou et al. present a new mean shift based FCM algorithm improving the computing cost. In [28], Melli et al. compare four color clusterization methods: median-cut, k-means, FCM and mean shift, the latter having obtained the best results. In [29], Celebi et al. carry out the segmentation using the JSEG algorithm based on the color quantization and spatial segmentation. In [30], Celebi et al. use the statistical region merging algorithm method comparing the results with six methods of the state of the art, with very good results. The use of the watershed technique has been proposed in some works; one of the most relevance is presented in [31], where Wang et al. use the watershed approach, and adding for the improvement of the segmentation a postprocess by a correction based on neural networks. The technique of the active contours/snakes is described in the methods section. This method is normally used in semiautomatic systems, which require some interaction by the user, although there also exist methods in which this interaction is not undertaken, like [32], in which Erkol et al. use a method based on gradient vector flow (GVF) snakes with an automatic initialization. More recently, in [33], Zhou et al. propose an improved algorithm based on GVF and, in [34], Abbas et al. present a work that uses the active contour model in the CIECAM02 based uniform color space, to achieve an adaptation to human perception. Detection and Characterization of Indicators and DiagnosisPreviously the most commonly used medical methods for the diagnosis were commented. But examining the indicators present in those methods, it can be observed that the objectivization of some of the present indicators, the C of the “Color” of the “ABCD Rule”, can be easily quantified and objectifiable. However, there exist other indicators, as it is the case of many of the patterns or dermoscopic structures, which are very difficult to quantify and objectify, in fact, its determination by human experts is largely subjective. Thus, many methods of the state of the art have other approach. Figures 5 and 6 show the two approaches, firstly the classical approach, that maps directly the detection and characterization of indicators from the medical algorithm, and secondly the other approach, where a process of extraction of features is undertaken and, on the basis of a classification procedure, the diagnosis is determined. ![]() Fig. 5 Diagnosis made with the classical approach mapping directly from the medical algorithm ![]() Fig. 6 Diagnosis made with the approach based on the extraction of features and classification algorithms In the second approach should be noted that, really, these features behave themselves like the indicators of the algorithm. Normally, this approach uses supervised machine learning techniques: based on a set of features, a sample of image values is extracted, and its numerical values are studied in relation with its benignity or malignancy. On the basis of this, some classification rules are generated, which determine the diagnosis, and which are applied over the entirety of images. The problem this approach has is that, these being habitually automations are a support for the diagnosis of the doctor and, since he is the one who takes the decision at the end, he usually prefers to do it supported by algorithms used in the medical practice, and that is why he feels more comfortable if the guide to the diagnostic shows him the estimated value of the medical indicators. Hence, the classical approach which is to be followed is to attempt to reproduce as closely as possible the medical method, making to do so a detection and characterization of the indicators of the medical algorithms. The system presented here would be located in this classical approach. We are watching some of the most relevant works, using both approaches. In [35], Iyatomi et al. carry out an implementation of the ABCD algorithm by selecting 80 features for the A, 32 for the B, 140 for the C and for the D, instead of undertaking the detection of different dermoscopic structures, it selects 176 texture features, and the process of machine learning and rule generations is made with ANN (Artificial Neural Network). In [36], Alcon et al. present a detection system based on the ABCD algorithm, with an approach similar to the previous one, selecting features for A, B, C and D, and taking texture features for the D. For the rule generations various classifiers are used. In [37], Di Leo et al. present a detection system based on the 7-Point Checklist Method. For the detection of the different structures they use color, texture, spectral and structural analysis features. The analysis and classification are made using statistical methods and machine learning methods. In [38], Ganster et al. select a set of color, geometric and texture features to describe the malignancy of a lesion, delivering the final kNN classification a sensitivity of 87 % with a specificity of 92 %. In [39], Tomassi et al. define a series of color and texture features, then a classification with SVM and spin glass-Markov random fields. The results are compared, over 270 images, with those of the previous method exposed by Ganster et al. In [40], Celebi et al. present a work in which a series of geometric features are defined, color and texture ones, which are subsequently studied with SVM, using kernel RBF. Out of a total of 564 images a sensitivity of 84.33 % and a specificity of 96.19 % are obtained. In [41], Rahman et al. present a system of detection of melanoma. 128 features of color and texture are presented, the classification is made with Bayes and 358 images are tested, with an accuracy of 75.69 %. In [42], Surowka presents a work in which supervised learning with MLP and SVM is used. The feature set is composed of wavelet-based multi-resolution filters of dermoscopy images, and feature selection is done by the Ridge linear models. A sensitivity of 89.2–94.7 % and a specificity of 85–95 % are obtained. In [43], Situ et al. use the technique of Bag-of-Features (BoF), widely used in artificial vision and equivalent to the Bag-of-Words used in the documents. The classification results are made using Naive Bayes classification and Support Vector Machines. The best performance obtained is 82.21 % on a dataset of 100 skin lesion images. In [44], Zhou et al. present a new technique for the detection and description of invariant rotation and scaling features. Based on the interest point detection model, the concept of DIP (Dermoscopic Interest Points) is introduced for the first time, and a detector and descriptor of those points is proposed, comparing the information taken with others of the state of the art like SIFT and SURF over 150 images, obtaining good results. In [45], Situ et al. present a work in which, using the same BoF framework designed in [43], implement lesion classification using DIPs. SVM classifier is used, and over 1505 images 86.17 % of accuracy and 84.68 % of specificity are obtained. Regarding the detection of the individual patterns, it will be commented hereafter. In [46], Anantha et al. use for the detection of the pigment network texture analysis algorithms. In [47], Betta et al. for the detection of the atypical pigment network use two techniques, one structural, in which morphological methods are used, and another one spectral, in which the FFT is used, high pass filters, inverse FFT and finally thresholding techniques. Furthermore, in this work, a pixel classification method is used to find the pixels belonging to a vascular pattern. In [48], Di Leo et al. from the same research group, improve the previous work, defining 9 chromatic characteristics and 4 spatial ones, and use decision tree classifiers, in the categories of “Absent”, “Typical” and “Atypical”, using the C4.5 algorithm. The process is carried out over 173 images with more than 85 % of sensitivity and specificity (the exact values are not specified). In [49], Barata et al. undertake the detection of the pigment network using a bank of directional filters and morphological operations, followed by a feature extraction and an AdaBoost algorithm for the classification, and then obtaining over a database of 200 images a sensitivity of 91.1 % and a specificity of 82.1 %. In [50], Shrestha et al. use different texture metrics for the analysis of the del atypical pigment network, with an accuracy of 95 %. In [51], Sadeghi et al. carry out the detection of the pigment network using the Laplacian of Gaussian (LoG) filter in the first place to capture correctly the “Light-Dark-Light” and then, over the generated binary image, seeks cyclical sub-networks, using the ILCA algorithm (Iterative Loop Counting Algorithm). 500 images are tested with an accuracy of 94.3 %. In [52], Sadeghi et al. from the same research group, improve the previous work and present a method for the classification between “absence of pigment network”, “typical pigment network” and “atypical pigment network”. To do so they propose an algorithm based on the previous work to find the mesh and the extraction of structural, geometric, chromatic and texture characteristics, treated with the LoginBoost algorithm, thereby generating a classification between “Absent”, “Typical” and “Atypical”, over 436 images. 82.3 % of accuracy is obtained. If it is made over the “Absent” and “Present” categories, 93.30 % of accuracy is obtained. In [53], Skrovseth et al. seek different texture parameters for the detection of the pigment network and the globular pattern. In [54],Yoshino et al. present a work in which morphological filters are used for the detection of the globular pattern. In [14], Gola et al. undertake the detection of globules combining morphological techniques with an edge detection algorithm. In [55], Celebi et al. study the presence of the pattern blue-white veil in the melanocytic lesions. To do so a series of features corresponding to the veil pixels are captured, and a tree for the classification is generated, using the C4.5 algorithm, with the rules obtained for the classification of the pixels of a skin lesion between veil and not veil. After that, the study about if the region with the pixels might have the blue-white pattern, on the basis of geometric features, using the C4.5, and generating a tree with the corresponding rules. In [56], Di Leo et al. carry out a partition of the lesion into different colors using PCA. Over the regions some features are defined, to determine those of blue-white veil and those of regression. LMT are used, generating a tree of rules, achieving sensitivity results of 87 % and specificity results of 85 %. In [57], Garcia et al. study the blue-white veil and the dark-red patch of pigment patterns in melanocytic lesions, using machine learning techniques, obtaining color features for the pixels detection and calculating morphological and geometric features for the regions classification, and also incorporating in the generated rules for the patterns detection the relation between both types of structures. For the streaks detection, in [58], Mirzaalian et al. develop a machine-learning approach, using for the identification of the streaks color, spectral and geometric features, and for the classification a SVM model, validating the method over 99 images, differenced in “absence”, “presence of regular” and “presence of irregular”, with an AUC of 91 %. In [59] Sadeghi et al. present a novel approach for the detection of the streaks, applying techniques based on ridge and valley detection used in fingerprint image recognition. It is tested in 300 images of melanocytic lesions, with an AUC of 90.5 %. In [60], Sadeghi et al. from the same research group, improve the previous work, and present a method for the detection of the streaks. They classify the dermoscopy images into streaks “Absent”, “Regular” and “Irregular”, testing in 945 images, and obtaining an AUC of 85 %. This method also includes the Absent/Present and Regular/Irregular classifications. For the detection of the hypopigmentation pattern, in [61], Dalal et al. study the features of the hypopigmentation areas from different point of views (color, geometry, position inside the lesion, etc.), and using neuronal networks arrive to a series of rules. For the detection of blotches, in [62], Madasu et al. present a work in which blotches are identified using the texture analysis. In [63], Stoecker et al. design a blotches extraction algorithm divided into two parts. First of all the thresholding is made to find the regions and, secondly, they are analyzed through a series of features, using machine learning techniques. Results with an accuracy of 77 % are obtained. For the detection of blotches, in [64], Pellacani et al. present two methods for the automated extraction of ’Absolute’ (ADAS) and “Relative” dark areas (RDA) and a set of parameters for its description. It is tested in 339 images of melanocytic lesions, sifting from melanomas and nevus, obtaining a result of 74.2 and 71.2 % respectively. In [65], Khan et al. use fuzzy techniques for the detection of blotches. A machine learning process is carried out, obtaining some rules, using neuronal networks. An accuracy of 81.2 % is obtained. For the detection of parallel pattern, typical in acral volar melanomas, in [66], Iyatomi et al. propose a novel method, obtaining over a dataset of 213 images a sensitivity of 81.1 % and a specificity of 92.1 %, consisting in three subpattern detectors for the three typical parallel structures such as parallel ridge, parallel furrow and fibrillar pattern. They use color, texture and structural features, reduced by using PCA, and classified by a linear model. Most Relevant Tools for the Automated Detection of Melanoma in Dermoscopic ImagesA state of the art of some of the most relevant software systems for the automated detection of melanoma in dermoscopic images will be carried out. To do so, first of all, a characterization of the automated systems in dermoscopic images will be undertaken, describing some of the criteria to be taken into account in those systems, secondly a brief description of the most relevant systems in the market will be made, and finally, thirdly, a comparison between them is carried out. Characterization of the Software Systems for the Automated Detection of Melanoma in Dermoscopic ImagesSome of the characteristics of interest which are used to be taken into account when characterizing a software system for the automated detection of melanoma in dermoscopic images will be carried out. It should be noted, in any case, that the approach taken in this review is to place especially the standpoint in the analysis of the software systems in support to the diagnosis used and it shall not be assessed the technical specifications of the hardware which in most cases are integrated with the software. Integrated with proprietary hardware: Most of the relevant systems work with proprietary hardware, having been developed by the manufacturers themselves, which complement the acquisition of images with a software to support to the diagnosis. The advantage of this is that it assures a stronger integration between the software system and the acquired images. The disadvantage is the coupling this may induce to, and the worse flexibility when analyzing the not acquired with proprietary technology. Teledermatoscopy: A system with teledermatoscopy support is capable of undertaking the distance transmission of dermoscopic images, for its remote analysis. The teledermatoscopy can be used with different purposes: for the medical user it allows to carry out the diagnosis from a remote center, or else a second diagnosis to review the diagnosis already carried out; for the non-medical user it allows the possibility of making the screening directly, via mobile or web, or else, it should be integrated in a telemedicine platform. Multiplatform: Quite related to the previous one, this characteristic has to do with the different platforms from which it can be accessed to the diagnostic system, via web, mobile devices, via web services, via desktop application, etc. Multiaccess: There exist systems which can be accessed by the public in general, other ones which can only be accessed by health professionals and other ones which allow both types of users. Bodymapping: Currently, most of the systems have access to the bodymapping, that is to say, they are capable of storing and processing macroscopic images corresponding to parts of the body, with sets of lesions, mapped to dermoscopic images corresponding to the different lesions. Notwithstanding, for the accomplishment of this task there exist different degrees of sophistication, which allow a greater agility in the procurement and processing of this information. Macroscopic images: Most of the systems of automated detection of melanoma are capable of analyzing macroscopic images of the lesion, carried out with a normal photo camera, generally without any magnification. Obviously, this analysis always has a worse accuracy, but in some occasions it can be convenient. Classic dermoscopic images: By “classic dermoscopic” images we mean the ones obtained through the utilization of a dermatoscope. Usually, these images are defined as just dermoscopic, however, here we have established the convention of encompassing the multispectral ones within the dermoscopic ones, and to differentiate them they will be named as “classic dermoscopic” . Multispectral dermoscopic images: Images obtained by a device using different wavelenghts of light, which penetrate the skin to different depths, which allow the visualization of invisible criteria to macroscopic images and classic dermoscopic ones. Various images in gray are obtained corresponding to the different bands on the spectrum which are handled. Frequently quoted and positively evaluated in relevant publications in recent years: When a system is quoted in a relevant publication is regarded as a system of greater acceptance by the scientific and medical community, being also important the assessment given to it. Obviously, there are always questionable systems of analyzing, depending on who carries out the experiment and how this is undertaken, the database tested, and so on. High degree of penetration on the market of the health professionals: It is an important characteristic the analysis of the degree of penetration on the market, among the health professionals, since if this is high it can be an indicator that the experts trust in it. In any case, it always has to be taken into account that, in many instances the hardware and the software are sold integrated and, consequently, the sale of the software might be due to the hardware. And, of course, many other commercial considerations can be taken. Approval by institutions: A key feature when it comes to assess the reliability of a diagnostic is its approval by the institutions. Algorithm for the diagnosis: Obviously, all the software systems implement proprietary algorithms. Notwithstanding, this automations are really a guide to the diagnosis of the doctor, and since he is the one who takes the decision at the end, in many instances he prefers to do so supporting in algorithms accepted by the medicine. Consequently, normally, the diagnosis is accompanied by the quantitative information corresponding to a medical algorithm. Accuracy: It is obvious that the most important feature of an automated system is its level of accuracy, and more specifically its sensitivity and specificity. Notwithstanding, it deals with a sensitive issue. Many corporations do not provide these data explicitly and, in any case, as it has been commented previously, these data need to be taken with great caution, since the accuracy data are always questionable data to analyze, depending on who does the experiment and how this was carried out, the database tested, whether the difficulty is higher or lower in the discernment of images, the factors to be taken into account, and so on. Most Relevant Automated Systems for the Detection of Melanoma on the MarketSome of the, in our opinion, most relevant software systems for the automated detection of melanoma in dermoscopic images will be commented here, based on the previously mentioned criteria and always from the point of view of the software in support of the diagnosis. Additionally, it should be also noted that, presently, due to the importance of the early detection of melanoma, the applications in macroscopic images aimed at the public in general are proliferating. To illustrate this type of tools, which are obviously less accurate, three systems which work exclusively with macroscopic images will be commented, two of them are mobile applications. Finally, the Molemap, the most important screening worldwide program for the detection of melanoma, and Melanoscan, a screening program which is having good results lately, will be commented. In both cases the diagnostic software is not commercialized per se but, nevertheless, due to its importance, it has been considered appropriate to include them here. As it has been stated previously, it should be noted that most of the relevant systems work with proprietary hardware, having been developed by the manufacturers themselves, which complement the acquisition of images with a software supporting the diagnosis though, in many cases, this was developed in principle by a research team external to the manufacturing company. MoleMax™It is a property of the Derma Medical Systems company (Austria) and it was developed in cooperation with the medical faculty of the University of Vienna [69]. It is a system which lasts present on the market for many years, and it works in classic dermoscopic images obtained with proprietary hardware. In fact, today MoleMax™ is a worldwide accepted clinical standard in digital epiluminescence microscopy. It also allows the analysis of macroscopic images, captured with digital cameras, and giving support for webcam. It is sold only to health professionals, although the interaction with the non-medical user system is allowed, for the accomplishment of screening and, in fact it is focused to teledermoscopy. It is multiplatform to a great extent, and it has been quoted and positively evaluated in various relevant publications. Its degree of penetration among the health professionals is very high. The diagnosis is undertaken in an automated way, using as diagnostic support the ABCD Rule and the 7-Point Checklist. Recent data about the accuracy of the Molemax diagnostic software are not available. It can be seen in Fig. 7. DBDermo-Mips/DDAXIt is a property of the Biomips company (Italy) [70]. It is a system for the aided diagnosis of melanoma which works in classic dermoscopic images, on the basis of the DBDermo-Mips system which remains for many years on the market. The main addendum is its great speed, which provides the possibility of performing in real-time analysis of the patient lesions, without the necessity of storing previously the images. It is not integrated with proprietary hardware, but there are dermatoscopes which it is more integrated with. It is only directed to health professionals, being its level of penetration between them high and having been quoted and positively evaluated in some relevant publications. The diagnosis is carried out using as medical algorithm the ABCD rule, although it analyses a large number of indicators using the same similarity pattern and the company itself reports an accuracy with a mean sensitivity of 92 % and a mean specificity higher than 80 % [70]. It can be seen in Fig. 8. FotofinderIt is a property of the FotoFinder Systems Inc. company (Germany) [13]. It is a system which works in classic dermoscopic images obtained with proprietary hardware. It is widespread among the health professionals being aimed only at them, offering a large number of performances, among them an advanced capture software for the bodymapping. It is multiplatform, allowing use even mobile devices integrating an optical device. It has been quoted and positively evaluated in various relevant publications. The diagnosis is made in an automated way using the diagnosis Mole Analyzer tool, having as diagnostic support the ABCD Rule. No recent data about the accuracy of the diagnostic Mole Analyzer software are available. It can be seen in Fig. 9. DermaGraphix/MirrorIt is a property of the Canfield Imaging Systems company (US) [71]. It is a system which works in classic dermoscopic images with proprietary hardware. It is quite established among health professionals, mainly in UK and US, and it has been quoted and positively evaluated in some relevant publications. The diagnosis is carried out in an automated way monitoring the evolution of the lesions on the skin and using for the diagnosis the ABCD rule. No relevant data about the accuracy of the diagnostic software is available. It can be seen in Fig. 10. SIAscopy™-MolemateIt is a property of the MedX Health Corporation company (Canada) [72]. It is a system which works in multispectral dermoscopic images obtained with proprietary hardware, which are generated using the SIAscopy™ technology, and they correspond to eight consecutive discrete wavebands between 400 and 1,000 nm penetrating to a depth of 2 mm. It is directed only to the health professionals, being high its level of penetration and having been quoted and positively evaluated in some relevant publications. The diagnosis is made in an automated way, using the Molemate tool, on the basis of proprietary algorithms. On July 2012, the British Medical Journal published a study in which Molemate obtained good results, though no evidence that the MoleMate system improved appropriateness of referral was found and, neither if the systematic application of best practice guidelines alone was more accurate than the MoleMate system [73]. It can be seen in Fig. 11.
![]() |