Database
Data elements
Discharge Abstract Database (DAD)
• All acute care hospitalizations in Ontario after 1991
• Information regarding the admitting hospital, admission diagnosis, in-hospital interventions and length of stay, diagnoses contributing to the hospital stay, and discharge disposition
National Ambulatory Care Reporting System (NACRS)
• All ambulatory visits, including emergency department and outpatient visits information regarding presenting complaint, interventions, triage category, and discharge disposition
Ontario Mental Health Reporting System (OMHRS)
• All admissions to a mental health facility in Ontario, starting in 2005 DSM-IV axis I and axis II diagnoses at admission and discharge marital and employment status
• Presence of specific psychiatric symptoms, information regarding self-harm attempts and their intent, and information regarding substance use
Registered Persons Database (RPDB)
• Demographic and vital statistics information date of last contact with healthcare system
Ontario Register General, Death (ORGD)
• Data on all deaths in Ontario date, location, and immediate, antecedent, and underlying cause of death
Research-specific indices can also be generated using these datasets and applied to future projects. For example, the Ontario Marginalization Index (ONMARG) was created by researchers at the Centre for Research on Inner City Health (now the Centre for Urban Health Solutions) in Toronto to facilitate exploration of how multiple dimensions of social marginalization are concentrated at the local level, and how these factors are associated with health outcomes [7]. ONMARG is derived from 2006 census data and includes four dimensions of marginalization: residential instability, material deprivation, ethnic concentration, and dependency. The ONMARG for individuals is derived based on their census subdivision (smallest geographical unit), dissemination area, or local health integration authority (largest geographical unit). As such, the ONMARG does not represent individual-level data.
Health administrative databases are maintained in various other forms worldwide. Similar datasets are maintained in Manitoba, Canada, by the Manitoba Centre for Health Policy. These data can be used to evaluate functioning of the healthcare system and interactions with other publicly funded systems: education, justice. Other countries with large health administrative databases include Australia, Israel, and Taiwan. In the United States, the Veteran Affairs Clinical Database contains medical claims-based data for all Veterans, and Medicaid contains medical claims-based data for all recipients of social welfare. In the United Kingdom, the Hospital Episode Statistics database contains information on all hospital visits that are funded by the National Health Service.
Health administrative databases differ from registries that are maintained for research purposes. The National Burn Repository is perhaps the largest and most well-known database of burn-injured patients. It is maintained by the American Burn Association and represents an amalgamation of data voluntarily submitted by burn centers. Most of these burn centers are located in the United States; the 2016 report of data contained information from 96 US burn centers, in addition to 4 Canadian, 2 Swedish, and 1 Swiss burn center, representing more than 205,000 entries [8]. Although the NBR represents a rich source of burn-specific data, studies using this data are not considered population-based. The voluntary nature of burn centers reporting data to the NBR means that the data are a convenience sample of the burn-injured population, and not necessarily a representative sample. Furthermore, the NBR does not allow researchers to examine trends in the incidence of burn injury at the population level over time, or to relate outcomes to population-level variables. For example, data in the NBR might suggest a decrease in burn-related hospitalizations over time; however, the denominator (i.e., number of population at risk) is unknown. Nonetheless, the NBR is able to provide robust estimates of changes in burn patient demographics, incidence of complications during hospitalization, length of stay, and burn injury characteristics.
5.3 Advantages of Population-Based Research
There are several advantages to conducting population-based research using large health administrative databases. By the nature of such datasets, information about the healthcare utilization for all individuals in a given population is collected and stored objectively and consistently over time [2]. This facilitates the capture of information about a large number of individuals over indefinite periods of time, both prior to and following a given date, thereby permitting use of longitudinal study designs, with minimal loss to follow-up. The ability to “look back” in time before a given event overcomes the recall bias that can be associated with asking study participants to recall prior healthcare use, and allows adjustment or exclusion based on prior healthcare utilization. In the case of relatively rare events such as burn injury, population-based databases allow identification and follow-up of a large sample of burn-injured individuals, spanning an entire geographic region. As a result, with a relatively short and inexpensive study design, researchers can generate data about incidence, trends, and outcomes related to a given exposure in an entire population, over defined study periods. To gather such information using traditional research methods would be costly, time-consuming, and would be subject to losses to follow-up.
Population-based study designs also facilitate the relatively simple identification of a number of control members from the general population, matched on any number of patient characteristics, including but not limited to age, sex, geographic residence, socioeconomic status, and medical comorbidities. Identifying such controls in a retrospective or prospective cohort study would be incredibly onerous. The use of a controlled study design allows the description of relative, rather than just absolute risks.
5.4 Limitations of Population-Based Data
5.4.1 Ascertainment of Disorders and Burn Injury Characteristics
While administrative data has tremendous strengths, it is also limited in a variety of ways. Specifically, data are limited by ascertainment bias, and are ultimately representations of individuals who sought or received care from a care provider or were hospitalized [9]. As such, these health indicators report treatment use. While due to their severity, burn treatment rates are likely to reflect accurate rates of injury, sequalae such as mental disorders or suicidal behavior associated with the injury may be limited to individuals that sought care [10, 11]. Failure to seek help for mental disorders is a problem worldwide; certain populations, including males and older individuals, are less likely to seek care [12]. As a result, mental disorder measures that rely on administrative data are likely underestimated. While many diagnoses are available through the use of administrative data, some measures such as post-traumatic stress disorder (PTSD) are not always captured due to ICD coding challenges. For example, it is not possible to differentiate PTSD from other anxiety conditions using data housed at the Manitoba Centre for Health Policy unless the patient is hospitalized. Similarly, other measures related to burn injury might not be recorded accurately using administrative data such as burn depth and location. As a result, it is not always possible to examine injury characteristics and associated outcomes. While this limitation is present, administrative data can be augmented by specialized clinical databases which contain more detailed information specific burn injury, as is being done in Manitoba [13].
5.4.2 Social Factors
Social factors play an important role in an individual’s health and response to injury [14, 15]. Factors such as social supports are an important component of recovery from injury, with increased perception of social support associated with improved quality of life post-burn injury [16]. Unfortunately, social measures including family supports following burn injury are not typically available in administrative data. Similarly, measures that may predict outcomes and quality of life among burn survivors including stigmatization, survivor guilt [17], and participation in burn survivor support groups [18] are also unavailable resulting in an incomplete picture of an individuals’ adjustment to injury.
5.4.3 Immeasurable Time Bias and Loss to Follow-up
Immeasurable time bias and loss to follow-up are two potential limitations associated with the use of administrative data in the study of burn injury. Specifically, immeasurable time bias is when a health outcome or exposure is not measurable in a given period (such as cardiac events following pediatric burn injury) [19]. In Manitoba, medication used during hospitalizations is not captured, and as such medication use is available for outpatient visits only. Similarly, individuals who move out of province or who die over the study period would not contribute equal follow-up time in longitudinal studies. As such, different methodological and statistical approaches such as Cox Proportional Hazards Regression or offsets using log of person years may be used to account for censoring of incomplete observations or to ensure that follow-up periods reflect an individual’s time at risk [20].
5.4.4 Lack of Randomization
While population-based data typically utilizes information from all individuals in a population, there is a lack of randomization. As such, the advantages of randomization including the assumption that known and unknown confounding factors are equal in both study and control groups and reduction of bias are not possible. While these limitations are present, both weighting and matching methods exist that may correct for selection biases in studies where random selection is not used. Specifically, propensity score matching (PSM) and inverse probability treatment weighting (IPTW) can be used to either create a composite score of selected covariates or assign more or less weight to individuals that have lower odds of being in a case or control group [21]. As such, case and control groups are equalized in the measures included in either the propensity score or weight. While these methods account for measurable confounders, it is important to recognize that unmeasured confounding factors are not accounted for, therefore residual confounding may impact estimates.
5.4.5 Health Indicator Coding
Health indicators available in administrative databases are vast. Databases may include vital statistics, hospital and medical claims, social services and education data and other registries [9]. While access to this data provides researchers and policymakers with tremendous opportunities, it is essential that defined, valid, and reliable health indicators are used. When using administrative data-derived indicators, it is essential to understand that data are collected for non-research purposes, including health system management and healthcare provider payments [22]. Therefore, investigators must carefully consider whether or not such indicators are appropriate measures of the variable of interest. Although some sources have cited the lack of data validation as a potential limitation of using administrative data in research [23], the reliability and validity of registries at the Manitoba Centre for Health Policy (MCHP) have been examined [24–26]. These studies provide support for the validation and utilization of measures related to the study of burn injury-related mental disorder outcomes, including measures of mood and anxiety disorder diagnoses [9]. A related limitation of using administrative data is how, or at what level, data are coded. In many cases, an individual may be coded as having either the presence or absence of disease (yes/no). When dichotomous outcomes or coding is present, it along with the research hypotheses will direct choice of statistical analyses.
5.4.6 Repeated Measures
As many administrative data studies use information that is collected over time, individuals will often contribute more than one data point over a study period. As a result, repeated measures are present. Such repeated data often violate the statistical assumption of independent observations [27]. Many methods exist to help account for these correlated (i.e., same individual) data. While analyses using administrative data are often complex and utilize multiple time points, it is essential to employ analyses that can accommodate the correlated nature of the data [28–31]. Paired t-tests and multivariate analysis of variance which are typically used for analyzing repeated measures may be limited in the analyses of such data [28, 31]. In this case, generalized estimating equations (GEE) facilitate regression analyses that take into consideration the correlated nature of complex data. GEE is an extension of generalized linear models and will ensure that correct inferences and estimates are produced [32].
5.5 Overcoming the Limitations Associated with Population-Based Data
5.5.1 Know Your Data
There are a number of ways that investigators can mitigate the limitations of using administrative datasets. Firstly, it is essential to have a thorough understanding of the methods by which their data is collected and coded, and the inherent limitations. This knowledge will facilitate an understanding of the limitations specific to a given project. For example, if using datasets that employ ICD-10 coding, investigators will be limited to this characterization of healthcare visits, which may not provide the granularity desired. Secondly, investigators should seek an understanding of the validity of the databases being used, including accuracy of coding. Many large administrative datasets will undergo validation studies on an ongoing basis in order to ensure data quality. For example, the Canadian Institute for Health Information (CIHI) utilizes a variety of measures to ensure accuracy and consistency in its databases [33]. In 2010, CIHI conducted a re-abstraction study which demonstrated 86% accuracy in reporting of the most responsible diagnosis for admission in the DAD [34]. Several other validation studies have demonstrated the accuracy of diagnoses codes in the DAD for the identification of inflammatory bowel disease , stroke, chronic obstructive pulmonary disease, and spinal cord injury [35–38].
5.5.2 Validation
Investigators planning to use health administrative databases in their research should consider conducting a validation study specific to their patient population of interest. To perform a validation study, a gold-standard dataset is required, against which the administrative data are compared [39]. This gold-standard may be derived from chart review, or from a pre-existing clinical dataset (such as the NBR). If a pre-existing clinical dataset is used, then its validity may have already been assured. This gold-standard dataset can then be compared against the administrative data to determine its validity. One such study was used to validate burn diagnosis codes in Ontario, Canada [40]. The authors utilized a prospectively maintained database from Canada’s largest burn center as their gold-standard. This database was linked to a cohort of burn-injured individuals identified in the administrative dataset using patient-specific identifiers. Briefly, that study found that TBSA codes were highly sensitive and specific in identifying patients with ≥10 and ≥20% TBSA injuries (89/93% sensitive and 95/97% specific), with excellent agreement (κ, 0.85/κ, 0.88). Codes were weakly sensitive (68%) in identifying ≥10% TBSA full-thickness burn though highly specific (86%) with moderate agreement (κ, 0.46). The diagnoses codes had limited sensitivity (43%) to identify inhalation injury, but high specificity (99%) with moderate agreement (κ, 0.54). Burn mechanism had excellent coding agreement (κ, 0.84).
The above-mentioned validation study provides some important insights into the limitations of using administrative data to study burn outcomes. For example, burn depth was not reliably reported, owing to limitations of the ICD-10 coding system, and inhalation injury was underreported in the administrative datasets. Burn size and mechanism were accurately coded in the administrative data, while codes pertaining to the location of the burn were infrequently used. Therefore, this particular dataset is limited in its ability to provide details of burn depth or location and the potential association of these injury characteristics with any outcomes of interest. The ability to risk-adjust outcomes for these particular variables is also limited. Whether the limitations of Ontario’s administrative databases are generalizable to other administrative databases is unknown; however, the limitations specific to ICD-10 coding are expected to be limitations of any database employing this coding structure.
5.5.3 Linkage with Other Datasets
To provide greater clinical granularity to administrative data, it may be possible to link a clinical database to an administrative dataset. Such an approach has been used to study burn injury in Manitoba. In this case, a specialized provincial burn database has been linked with administrative data, and enables detailed study and follow-up [13].
Such linkage can also overcome any challenges associated with identifying a specific cohort of burn-injured individuals in an administrative database because the cohort can be identified in the clinical database and then followed over time in the administrative dataset after linkage occurs. Records can be linked either deterministically through the use of patient identifiers, or probabilistically using various algorithms. A combination of deterministic and probabilistic linkage is also possible. The exact nature by which records can be linked, and how the data are stored, will depend on the specific privacy and data sharing regulations of the administrative database.
Successful linkage of a clinical database to an administrative dataset will allow investigators to answer a number of research questions, as both burn-specific clinical data as well as long-term healthcare utilization data will be available. In a sense, such a dataset combines the best of prospective cohort studies and large population-based studies, without the time, expense, and loss to follow-up that might be associated with prospective studies. However, the logistics and cost of generating such a linked dataset should not be underestimated and will vary from region to region. Linkage also offers an opportunity to validate the administrative data, using the clinical database as a gold-standard, as discussed above.
5.6 Population-Based Studies of Burn Injury
Population-based studies have not yet been widely used in burn care research. However, much of the long-term outcome data available in the burn literature has been derived from population-based studies, mainly in Canada, Australia, and Taiwan. These countries have in common a publicly funded healthcare system; therefore large, healthcare administrative databases are maintained for the purposes of tracking healthcare utilization of all individuals eligible for coverage.
Some of the first population-based burn research was conducted in Australia, using the Western Australia Data Linkage System [41]. Using this dataset, Fiona Wood and colleagues derived a cohort of all individuals admitted to hospital for burn injury in Western Australia, between 1983 and 2008. These data facilitated the description of the epidemiology of burn injury in Australia and demonstrated a decrease in burn-related hospitalizations and mortality over time [41]. This group went on to match burn survivors by age and sex to non-injured members of the general population to determine whether rates of late mortality and specific types of hospital admissions are higher among burn survivors. These studies demonstrated increased late mortality among childhood, adolescent, adult, and elderly burn survivors [42–44]; increased hospitalizations for cardiovascular diseases, infectious diseases, diabetes, gastrointestinal disease, and nervous system disease [45–49]. Their work clearly illustrates the advantages of population-based research for burn injury: long-term follow-up, the ability to match to members of the uninjured population, and the ability to characterize temporal trends in burn incidence and mortality, while identifying groups that remain at high risk, to whom prevention efforts should perhaps be targeted.
In Taiwan, investigators have leveraged the availability of population-based datasets to conduct both descriptive and matched cohort studies. They have used these datasets to describe the epidemiology and associated healthcare utilization of burn injury in Taiwan, including the outpatient burden of burn injury; they found that only 3.6% of all burn-injured patients were hospitalized for treatment [50]. Three matched cohort studies have demonstrated that burn survivors are at increased risk of ischemic stroke after burn although the absolute risk is quite low [51–53].
In Canada, investigators have similarly used population-based datasets to describe the epidemiology of burn injury and to characterize changes in regionalization of burn care over time [54]. An advantage of population-based datasets is the ability to study patients treated at both burn and non-burn centers and to compare their outcomes. In one Canadian study, the investigators found that burn-related mortality had improved significantly over time at burn centers, with significantly more variation in mortality rates at non-burn centers. Furthermore, in 2013, more than 25% of patients with major burn injury received their care at non-burn centers. This highlights some of the insights that can be gained using a population-based approach. These datasets have also been used similarly to those in Taiwan and Australia to infer long-term outcomes from healthcare utilization data. In one such study, readmissions and emergency department visits were common after burn injury, most often related to mental illness and unintentional injuries, while burn recidivism was rare [55]. Interestingly, this study demonstrated that burn center care was associated with significantly fewer emergency department visits and readmissions.
Finally, two Canadian population-based datasets to characterize the association between burn injury and mental illness. In one longitudinal matched cohort study, Logsetty et al. found high rates of psychopathology among burn patients both before and after their injury, compared to a control cohort [13]. Their study highlighted the importance of mental healthcare for burn-injured patients, and the potential role that pre-existing mental illness might have on burn outcomes. Mason et al. utilized an exposure-crossover design to conduct their longitudinal cohort study, therefore allowing each burn patient to act as their own control before and after injury. This study demonstrated high rates of mental illness both before and after burn injury, similar to the results of Logsetty et al. While the overall rate of mental illness did not increase after burn injury, patients with minimal pre-burn mental illness experienced significant increases in their rate of mental health emergencies after burn [56]. This study also demonstrated that self-harm risk doubles after burn injury, underscoring the potential role for screening for mental health disorders during burn follow-up.
The use of administrative data also allows creation of unique study design that would be very difficult in clinical studies. An example of this is evaluation of parents of pediatric burn survivors [57]. Using administrative data, it is possible to create a cohort of injured children, a cohort of controls (uninjured children), identify the parents from each cohort, and evaluate the mental health not only post child’s injury, but also prior, thereby establishing if the rate of change in mental health caused by the injury is different from the control population. The obstacles in conducting this study, from identification of participants, consenting, dropout, and recollection bias would be insurmountable.
5.7 Conclusion
The studies discussed above offer only a small glimpse into the burn research possibilities afforded by the use of population-based datasets. As the focus of burn research shifts towards the measurement, evaluation, and improvement of long-term outcomes, both physical and psychological, population-based research will become an invaluable source of long-term outcome data for the burn investigator. The linkage of clinical databases, such as the NBR or other local registries, to population-based databases represents a powerful opportunity to study burn outcomes over both the short and long term, with the ability to generate comprehensive risk adjustment models, large sample sizes, long-term follow-up, and the ability to track and evaluate care provided both within and outside of burn centers. This knowledge will ultimately allow the creation of targeted interventions and care for individuals with burn injuries based on best evidence.
Summary Box
Population-based research offers an opportunity to follow a large cohort of individuals over time and measure rates of healthcare utilization at the population level.
This approach can be limited by a lack of clinical granularity in the data.
Validation and linkage to other datasets can overcome these limitations.
Many studies have successfully used a population-based approach to describe various outcomes after burn injury.
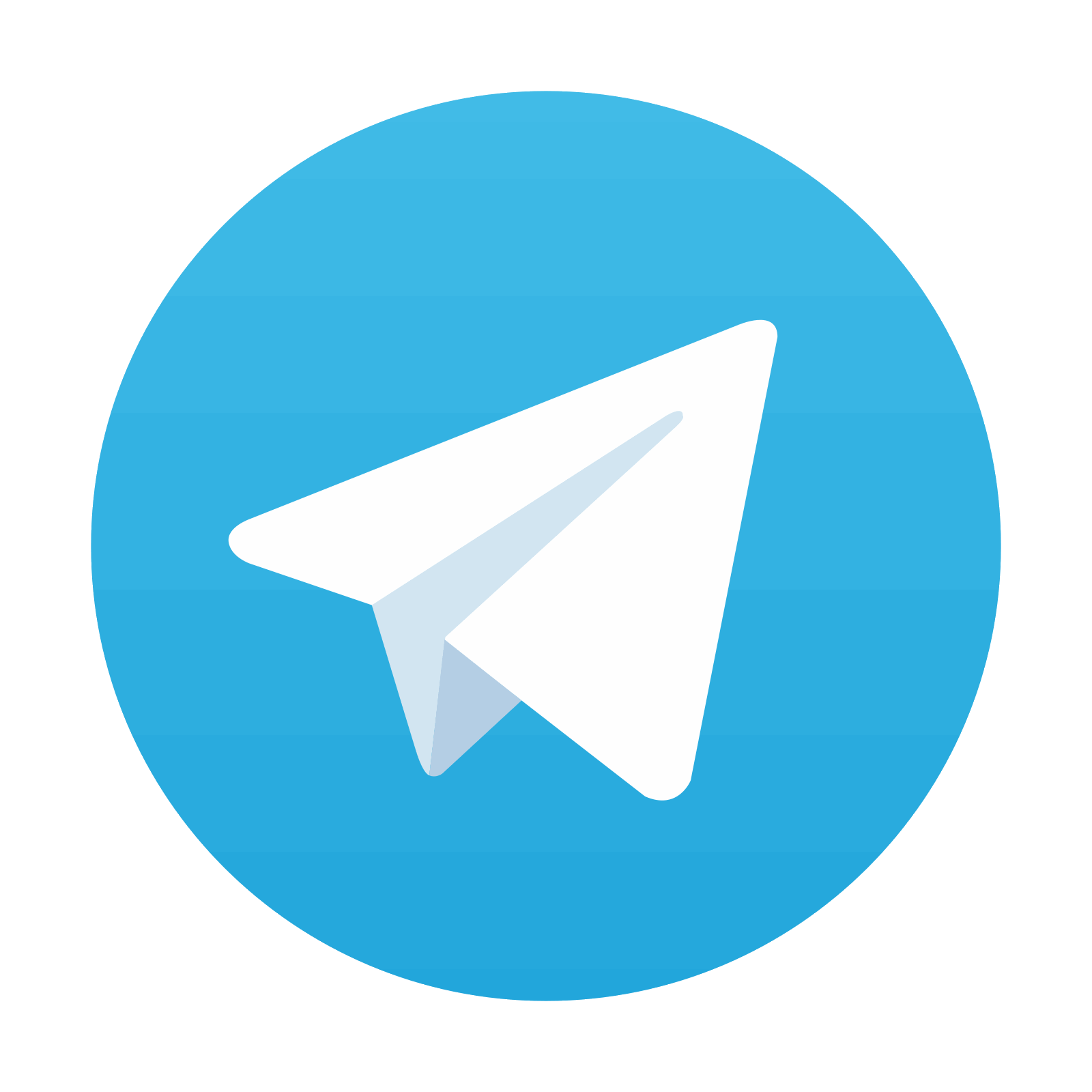
Stay updated, free articles. Join our Telegram channel

Full access? Get Clinical Tree
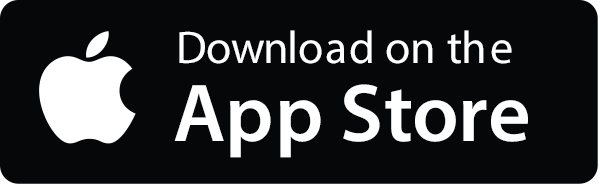
