Early diagnosis and treatment of melanoma improve survival. New technologies are emerging that may augment the diagnosis, assessment, and management of melanoma but penetrance into everyday practice is low. In the current health care climate, greater emphasis will be placed on the incorporation of technology for clinically suspicious pigmented lesions to facilitate better, more cost-effective management.
Key points
- •
Early diagnosis and treatment of melanoma improve survival.
- •
New technologies are emerging that may augment the diagnosis, assessment, and management of melanoma but penetrance into everyday practice is low.
- •
In the current health care climate, greater emphasis will be placed on the incorporation of technology for clinically suspicious pigmented lesions to facilitate better, more cost-effective management.
Introduction
Currently, a vast majority of dermatologic diagnoses are made by visual inspection alone and/or dermoscopy, without the opportunity to enhance clinical examination with imaging or molecular tests. Several newer technologies, however, are available, Food and Drug Administration (FDA) approved, and proved efficacious aiding in the diagnosis and prognosis of melanoma.
Despite the growing magnitude and dynamic evolution of research in this area, a recent survey reported only 11% of dermatologists even sometimes incorporate these emerging technologies into routine pigmented skin lesion (PSL) evaluation. Given this low penetration, there seems to be a significant lack of knowledge related to these technologies and/or a material lack of understanding of how to appropriately integrate them into clinical practice. To help fill this knowledge gap and help clinicians better understand the rationale for and appropriate utilization of these new tools, the 11-member Melanoma Evolving Diagnostic Technologies Integration Group (MEDTIG) ( Table 1 ) derived an algorithmic approach to systematically facilitate incorporating these technologies into the evaluation and management of suspicious PSLs. This algorithm is designed to serve as a template for clinicians to facilitate appropriate integration of these diagnostic and prognostic aids into their routine clinical practice.
Panel Participant | Affiliation |
---|---|
Richard R. Winkelmann, DO | Cutaneous Oncology Clinical Research Fellow, National Society for Cutaneous Medicine, New York, NY |
Clay J. Cockerell, MD | Clinical Professor of Dermatology, University of Texas Southwestern Medical Center, Dallas, TX |
Arthur J. Sober, MD | Professor of Dermatology, Harvard Medical School, Boston, MA |
Daniel M. Siegel, MD, MS | Clinical Professor of Dermatology, SUNY Downstate, Brooklyn, NY |
Sancy A. Leachman, MD, PhD | Chair, Department of Dermatology, Oregon Health & Science University, Portland, OR |
Whitney A. High, MD, JD | Associate Professor of Dermatology & Pathology, University of Colorado Denver School of Medicine, Aurora, CO |
Orit Markowitz, MD | Assistant Professor of Dermatology, Mount Sinai Medical Center, New York, NY |
Brian Berman, MD, PhD | Voluntary Professor of Dermatology, University of Miami Miller School of Medicine, Miami, FL |
David M. Pariser, MD | Professor of Dermatology, Eastern Virginia Medical School, Norfolk, VA |
Gary Goldenberg, MD | Assistant Clinical Professor of Dermatology, Mount Sinai Medical Center, New York, NY |
Theodore Rosen, MD | Professor of Dermatology, Baylor College of Medicine, Houston, TX |
Darrell S. Rigel, MD, MS | Department of Dermatology, New York University School of Medicine, New York, NY |
Introduction
Currently, a vast majority of dermatologic diagnoses are made by visual inspection alone and/or dermoscopy, without the opportunity to enhance clinical examination with imaging or molecular tests. Several newer technologies, however, are available, Food and Drug Administration (FDA) approved, and proved efficacious aiding in the diagnosis and prognosis of melanoma.
Despite the growing magnitude and dynamic evolution of research in this area, a recent survey reported only 11% of dermatologists even sometimes incorporate these emerging technologies into routine pigmented skin lesion (PSL) evaluation. Given this low penetration, there seems to be a significant lack of knowledge related to these technologies and/or a material lack of understanding of how to appropriately integrate them into clinical practice. To help fill this knowledge gap and help clinicians better understand the rationale for and appropriate utilization of these new tools, the 11-member Melanoma Evolving Diagnostic Technologies Integration Group (MEDTIG) ( Table 1 ) derived an algorithmic approach to systematically facilitate incorporating these technologies into the evaluation and management of suspicious PSLs. This algorithm is designed to serve as a template for clinicians to facilitate appropriate integration of these diagnostic and prognostic aids into their routine clinical practice.
Panel Participant | Affiliation |
---|---|
Richard R. Winkelmann, DO | Cutaneous Oncology Clinical Research Fellow, National Society for Cutaneous Medicine, New York, NY |
Clay J. Cockerell, MD | Clinical Professor of Dermatology, University of Texas Southwestern Medical Center, Dallas, TX |
Arthur J. Sober, MD | Professor of Dermatology, Harvard Medical School, Boston, MA |
Daniel M. Siegel, MD, MS | Clinical Professor of Dermatology, SUNY Downstate, Brooklyn, NY |
Sancy A. Leachman, MD, PhD | Chair, Department of Dermatology, Oregon Health & Science University, Portland, OR |
Whitney A. High, MD, JD | Associate Professor of Dermatology & Pathology, University of Colorado Denver School of Medicine, Aurora, CO |
Orit Markowitz, MD | Assistant Professor of Dermatology, Mount Sinai Medical Center, New York, NY |
Brian Berman, MD, PhD | Voluntary Professor of Dermatology, University of Miami Miller School of Medicine, Miami, FL |
David M. Pariser, MD | Professor of Dermatology, Eastern Virginia Medical School, Norfolk, VA |
Gary Goldenberg, MD | Assistant Clinical Professor of Dermatology, Mount Sinai Medical Center, New York, NY |
Theodore Rosen, MD | Professor of Dermatology, Baylor College of Medicine, Houston, TX |
Darrell S. Rigel, MD, MS | Department of Dermatology, New York University School of Medicine, New York, NY |
Methods
A Medline search was performed for keywords (Melanoma, Diagnosis, Technology, Dysplastic Nevi, Genetic Testing, Prognosis, Imaging, and Molecular Testing) and 806 abstracts from January 1, 2012, to June 15, 2015, were considered. Additional earlier articles were selected when directly relevant to the scope of this article. After subsequent review, 49 articles of technologies with FDA approval and evidence-based efficacy were selected based on Oxford Centre for Evidence-Based Medicine criteria and distributed to MEDTIG members. At a meeting on January 17, 2015, MEDTIG reviewed the best evidence pertaining to available methods for guiding decisions to biopsy suspicious PSLs, specimen evaluation, and melanoma risk stratification. Technologies that were not widely available, lacking support by strong translational studies, not FDA-approved, and/or in the process of validating their efficacy for melanoma diagnosis were not considered for inclusion in the algorithm. Technologies and their rationale for clinical utility have been described previously. A summary of their mechanisms, efficacy, clinical utility, and estimated costs is presented in Table 2 . The algorithm was developed by consensus using a modified Delphi technique. The Delphi technique has been effectively used in developing dermatologic expert panel recommendations to reach consensus. Using this approach, a minimum of 70% agreement among participants was required to adopt a proposal. If 70% agreement could not be achieved, the proposal was returned to the group to be modified until the 70% agreement was achieved. After the adoption or rejection of each proposal, the algorithm was modified in real time and subject to discussion over the course of many rounds to reach consensus.
Method/Technology | Purpose | Description | Clinical Validation | Interpretation | Clinical Impact | Practical Considerations | Estimated Cost b | Level of Evidence |
---|---|---|---|---|---|---|---|---|
Clinical examination | Identify suspicious PSLs with naked eye | Full-body skin examination | Sensitivity: 69% Specificity: 94% | Subjective | First step in evaluating PSLs for risk and consideration for biopsy |
| I | |
Dermoscopy | Evaluate suspicious PSLs | Uses 10× magnification with or without polarized light → pattern analysis | Sensitivity: 79%–93% Specificity: 69%–89% | Subjective | Studies have shown increased sensitivity and specificity for detection of melanoma compared with naked-eye examination |
| $300–$500 per device purchase | I |
MSDSLA | Provides additional clinical information to enhance clinician biopsy decisions | Computerized imaging and analysis of 10 multispectral bandwidths (430–950 nm) up to 2.5 mm below the skin surface to measure degree of PSL morphologic disorganization | Sensitivity: 62%–98% Specificity: 10%–63% | Objective | Aggregated data showed improvement in sensitivity, specificity, biopsy accuracy, negative predictive value, and positive predictive value with a decreased number of unnecessary biopsies |
| $100–$200 per sitting | II |
RCM | In vivo imaging allows for diagnosis and risk stratification | Uses a near-infrared 830-nm laser to image horizontal sections up to 300 μm in depth. Images can be captured sequentially to create 8 mm × 8 mm mosaic squares in a single horizontal plane at multiple levels of the epidermis and superficial dermis | Sensitivity: 91%–97% Specificity: 68%–86% | Subjective | Has been shown to increase sensitivity, specificity, and biopsy accuracy compared with dermoscopy and clinical examination |
| $100 per lesion interpretation | I |
Histopathology | Aids in diagnosis and staging | Method of staining and visualizing biopsied tissue under the microscope for interpretation by a dermatopathologist | No generalized systematic validation Favorable outcome accurately predicted in 47% of cases, unfavorable outcome accurately predicted in 73% of cases | Subjective | Histopathologic assessment of melanoma is required for AJCC staging. It is extremely unlikely that a vast majority of institutions and insurers would proceed with care for melanoma in the absence of a histopathologic diagnosis, rendered and signed, by a licensed physician. |
| $75–$150 per specimen | I |
IHC a | Adjuvant to histopathology for diagnosis of MM in equivocal PSLs | Visualization of antigens on biopsied tissue specimen using catalyzed enzymes conjugated to antibodies | Sensitivity: 69%–100% Specificity: unknown | Objective/ subjective | In PSLs with ambiguous or challenging histologic features IHC techniques may provide additional data to guide a final histologic interpretation |
| $56–$100 per stain | II |
aCGH | Adjuvant to histopathology for diagnosis of MM in equivocal PSLs | Method of analyzing copy number variations between entire genome of biopsied tissue and normal tissue controls | Sensitivity: 92%–96% Specificity: 87%–100% | Objective | In lesions with ambiguous histologic features aCGH may provide additional data to guide management |
| $1200–$1800 per specimen | II |
FISH | Adjuvant to histopathology for diagnosis of MM in equivocal PSLs | Allows identification of gene abnormalities on individual chromosomes typically using a 4-probe set with an internal control | Sensitivity: 43%–100% Specificity: 29%–80% | Subjective | In lesions with ambiguous histologic features, FISH may provide additional data to guide management |
| $600–$1000 per specimen | II |
Dx23-GEP | Adjuvant to histopathology for diagnosis of MM in equivocal PSLs | Gene expression profiling using qRT-PCR to evaluate expression signature of 23 genes differentially expressed in malignant and benign PSLs | Sensitivity: 89%–90% Specificity: 91%–93% | Objective | In lesions with ambiguous histologic features Dx23-GEP may provide additional data to guide management |
| $150 cost to patient | II |
Pr31-GEP | Assesses risk of metastasis in patients already diagnosed with MM | Gene expression profiling of isolated RNA via qRT-PCR measuring expression of 31 biomarkers within the tumor to classify stage I or II melanomas as low-risk (class 1) or high-risk (class 2) for future metastasis | Class 1 (lower metastatic risk subset): 79%–97% 5-y DFS Class 2 (higher metastatic risk subset): 31%–34% 5-y DFS | Objective | Provides prognostic information for stages I and II melanomas. May identify persons at risk for metastatic disease independent of AJCC stage, Breslow depth, ulceration status, mitotic rate, and SLNBx status |
| $200 cost to patient | II |
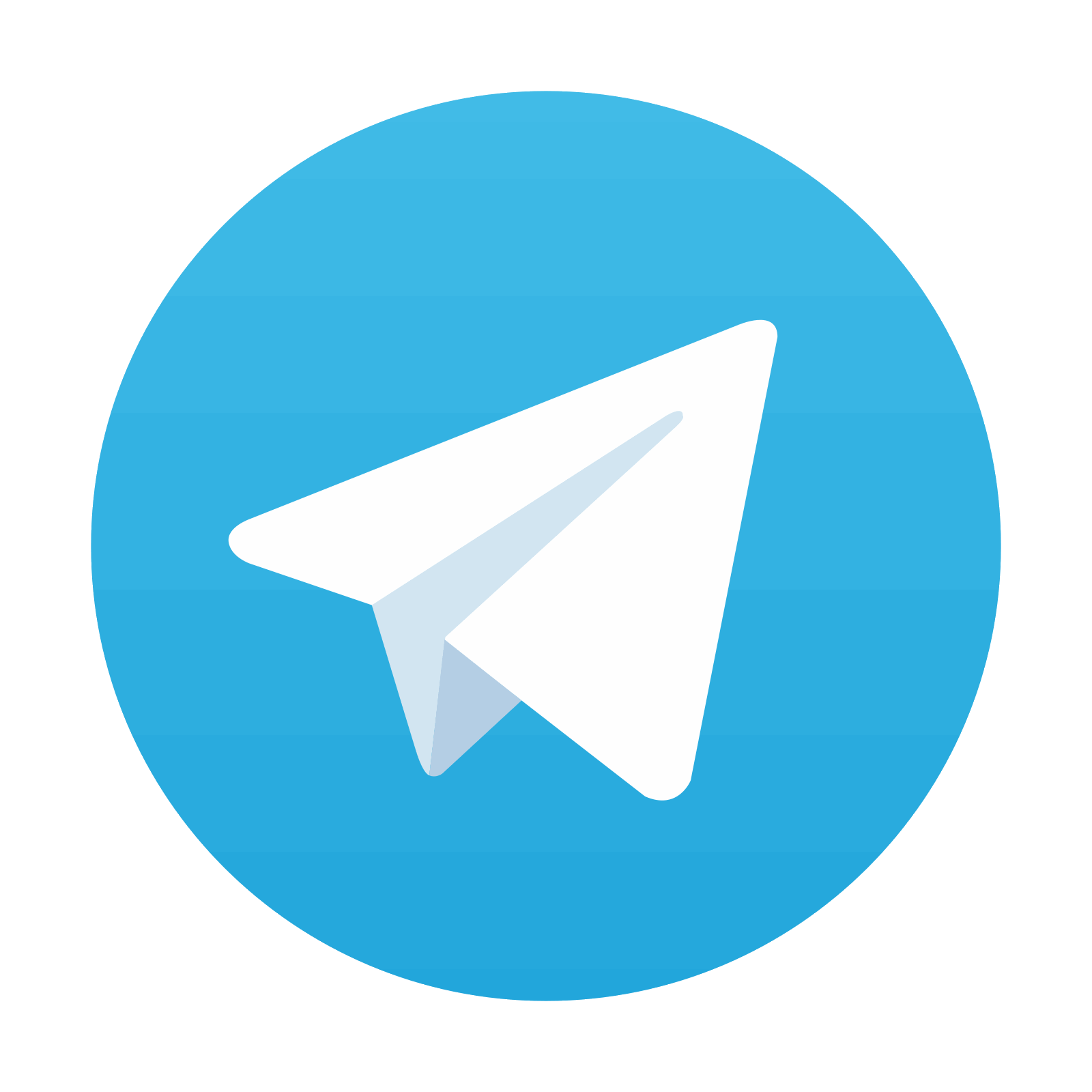
Stay updated, free articles. Join our Telegram channel

Full access? Get Clinical Tree
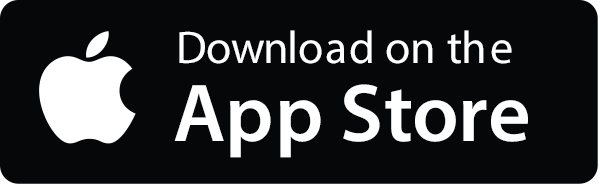

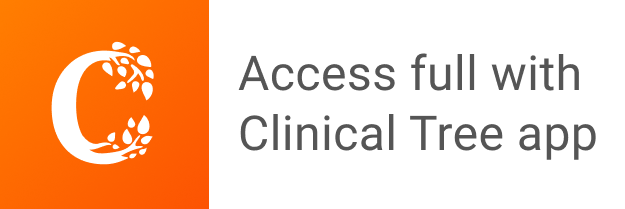