10 Evidence-based medicine and health services research in plastic surgery
Synopsis
The key take-home messages from this chapter are how to:
gather existing evidence on outcomes
interpret evidence on outcomes
design new studies to add evidence on outcomes
identify and analyze existing sources of data to create new evidence on outcomes
capture the patients’ perspective on outcomes
recognize the importance of an essential dimension of evidence: the patients’ perspective
consider the cost dimension in achieving outcomes
understand the challenges and approaches in translating good evidence into daily clinical practice.
The best evidence – where do we find it?
There are two ways to obtain best evidence for decision-making to improve the quality of care (Fig. 10.1). The first is to evaluate existing data and identify those reports that are convincing based on a good study design and clinically meaningful outcomes. When the current evidence is inadequate, the second choice is to produce new credible evidence. This section will describe a structure for evaluating existing evidence and then introduce methods for producing best evidence.
Evaluating existing literature
Best evidence requires three things: (1) a good study design; (2) an appropriate statistical analysis; and (3) clinically meaningful outcome measures. In a thorough review, Offer and Perks1 have enumerated the challenges that plastic surgeons face when trying to practice evidence-based medicine. They cite the lack of quality evidence in our literature as a major factor. Even a superficial perusal of our journals makes it clear that they are replete with descriptions of procedures by individual surgeons and the “outcomes” of those procedures in that surgeon’s hands. These studies, known as case reports or case series, have limited applicability to other practices, and are not structured, not compared, and not randomized. This is not only weak evidence on which to make medical decisions, but it is not an acceptable way to make such decisions in this era of healthcare reform and pay for performance (P4P).2 As shown in Figure 10.1, the stronger the evidence, the better the care one is able to deliver.
Literature search strategies
Practicing evidence-based medicine involves searching for the best available research, appraising the relevant studies for validity, and then translating the evidence to optimize patient care.3 Many clinical questions remain unanswered because of problems formulating relevant questions, insufficient access to information resources, and a lack of search skills.4 Today, there are a variety of strategies and web-based resources that allow searches of the relevant literature to answer many clinical questions.4 Some examples are provided below.
PubMed
The US National Library of Medicine provides access to more than 16 million citations through PubMed, accessing references from MEDLINE and directly from journals.4 Information from PubMed searches has been shown to improve both patient care and health outcomes significantly.4
Clinical Queries
Clinical Queries is a feature of PubMed that can help identify citations with the study design of interest. It is able to link the type of question (e.g., intervention, diagnosis, natural history, and outcome) to a search strategy that specifies the desired study design.4
PICO
The critical first step in the pursuit of evidence-based medicine is to ask a well-formulated question.4 Without sufficient focus and specificity, an otherwise relevant and important clinical question can be mired in irrelevant evidence.4 When the PICO framework is used with the PubMed Clinical Queries (see above), it has been shown to improve the efficiency of the literature search.4 The acronym PICO stands for patient problem, intervention, comparison, and outcome, and is a strategy to pose a well-formulated question.4 In a literature search for evidence in plastic surgery, for example, a question framed using the PICO approach might be expressed this way: In patients undergoing breast reduction (P), preoperative antibiotic prophylaxis (I) versus no prophylaxis (C), what is the rate of postoperative infections (O)?
Meta-analysis
Conceptually, a meta-analysis is the summation of multiple qualitative studies in order to increase the sample size, thus strengthening the conclusions over that which can be drawn from an individual article. This analysis allows researchers to reach a more reliable conclusion when there are conflicting results from multiple studies.5 Ideally, a meta-analysis is conducted using the highest level of evidence, such as randomized controlled clinical trials. Although meta-analysis can also be conducted on cohort studies and even case series, the quality of the evidence and, therefore, the conclusions are weaker.
The technique of meta-analysis may have limited applicability in plastic surgery, where there are few randomized trials and the metrics from one study to another may vary considerably. An example of a meta-analysis can be seen in Figure 10.2.6 This figure demonstrates an analysis of randomized trials evaluating the impact of tamoxifen on survival. As the reader can observe, there are multiple studies, with the findings of each plotted on a central axis. The summary analysis, which incorporates data from these studies, appears at the bottom of the vertical axis and suggests a benefit for the use of tamoxifen in early breast cancer. As can be seen, the 95% confidence interval for that summary measure is quite narrow. This implies a better point estimate of the true benefit of tamoxifen. Also note that the quality of the individual study is used to determine its weight in computing the summary score.
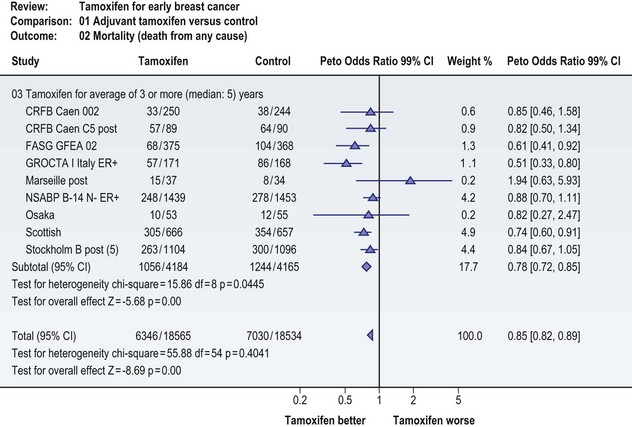
Fig. 10.2 Meta-analysis of randomized controlled trials evaluating the impact of adjuvant tamoxifen on survival risk among women with early breast cancer.6 Multiple studies have been included, with the findings of each plotted on the central axis. Data are represented as an odds ratio. (Odds ratio, similar to relative risk, reports the proportion of an occurrence to a nonoccurrence.) The summary analysis, which incorporates data from these studies, appears at the bottom of the vertical axis and suggests a benefit for the use of adjuvant tamoxifen in early breast cancer.
More recently, researchers conducted a meta-analysis of randomized controlled trials comparing rates of capsular contracture using either smooth or textured implants. These authors identified seven randomized controlled trials that addressed this issue. Individually four of the seven studies did not find a statistically significant decrease in the rate of contracture with textured implants. However, when the results of the studies were pooled, the meta-analysis suggested that textured implants had lower rates of contracture.7 To see further examples of good-quality meta-analyses, the reader is referred to the online Cochrane reviews.8
Systematic reviews
Systematic reviews are evaluations of the literature conducted according to clearly stated, scientific research methods that are designed to minimize the risk of bias and the errors associated with traditional literature reviews. The review process includes a comprehensive search based on defined criteria and includes a thorough evaluation of the quality and validity of the studies identified in the search process.9
The best-known source for systematic reviews is the Cochrane Database of Systematic Reviews, which contains over 3600 completed reviews.8 The Cochrane Group also provides a handbook for systematic reviews of interventions which prescribes seven steps for preparing a review:
2. identifying possible studies for inclusion
3. evaluation of selected studies
4. data collection and synthesis
Systematic reviews are influential tools in supporting evidence-based practice and some consider them to provide stronger evidence than randomized controlled trials. Moreover, they are essential for summarizing existing data, thereby avoiding the wasted effort that would result from unnecessary duplication of previous studies.9 To see further examples of good-quality systematic reviews, the reader is referred to the online Cochrane reviews.8
Study design and levels of evidence
Broadly, study designs can be divided into experimental and observational studies. Experimental studies, which include randomized controlled clinical trials, patient preference trials, and large, multicenter trials, test a hypothesis by examining the impact of an intervention on the outcome. In contrast, observational studies, which include cohort studies, case-control studies, case series, and case reports, describe the natural history or incidence of disease or analyze associations between risk factors and the outcomes of interest. As one might expect, there is generally a direct correlation between the complexity of a study design and the quality of the resulting data (Table 10.1).
Level of evidence | Type of study | Key features |
---|---|---|
Level I | Randomized controlled trial | Two randomly selected groups, one serving as a control, with a blinded comparison of outcomes of an intervention |
Level II | Prospective and retrospective cohort studies | A single group followed over time and evaluated for risk factors relative to outcome or incidence of disease |
Level III | Case-control study | Two groups, one with and one without disease, with a comparison of risk factors relative to outcomes |
Well-designed clinical study | Experimental study design with an intervention and a control arm compared for outcomes | |
Level IV | Cross-sectional cohort study | A single group evaluated at a single point in time for disease prevalence or risk factors |
Case series | A single group of consecutive cases identified by a disease or condition and followed over time | |
Level V | Expert opinion | Opinion based on experience and review of literature |
Case reports | A small group identified by a disease or condition and followed over time |
Adapted from the American Society of Clinical Oncology.
Experimental studies
Randomized controlled clinical trials
According to the National Institutes of Health, clinical trials are conducted in four phases, each serving a different purpose and helping researchers answer different questions (Table 10.2). In phase I, an experimental drug or intervention is evaluated in a small number of test subjects (20–80) to evaluate its safety, determine a dose–response curve, and identify potential side-effects. In phase II, the experimental drug or intervention is given to a larger group of subjects (100–300) to test its effectiveness and further evaluate its safety profile. In phase III, the experimental drug or intervention is given to a much larger group of subjects (1000–3000) in order to “confirm its effectiveness, monitor side-effects, compare it to commonly used treatments, and collect information that will allow the experimental drug or treatment to be used safely”. In phase IV, postmarketing surveillance is continued to identify additional information on the risks, benefits, and optimal use of the intervention.10
Table 10.2 Four phases of clinical trials
Phase | Purpose |
---|---|
I | To test an experimental drug or treatment in 20–80 people to evaluate safety, determine a safe dosage range, and identify side-effects |
II | To test the experimental drug or treatment in 100–300 people to determine effectiveness and evaluate its safety |
III | To test the experimental drug or treatment in 1000–3000 people to confirm effectiveness, monitor side-effects, compare it to commonly used treatments, and collect information to allow for its safe use |
IV | Postmarketing studies to learn more about the drug’s or treatment’s risks, benefits, and optimal use |
Unfortunately, randomized controlled trials are expensive and often impractical to answer questions that compare one surgical intervention to another.11,12 While patients are often willing to be randomized to take a pill versus a placebo, few are willing to be randomized to one of two surgical procedures or to a sham procedure. Further, surgeons often have a strong preference for one type of surgery over another and are therefore unwilling to randomize patients.13
Although the randomized controlled clinical trial is considered the “gold standard,” it is not without limitations.11 For example, the underlying assumptions of randomized controlled clinical trials may be invalid.11 The preferable treatment between two alternatives may be unclear; evidence of treatment effects from other sources may be insufficient; only specific effects resulting from the intervention are therapeutically valid; and only when the trial’s inclusion and exclusion criteria match the characteristics of an individual patient can the outcome of the study be fully transferred to clinical practice.11 In addition, randomized controlled clinical trials are inadequate to study infrequent or delayed adverse events; they are difficult, if not impossible, to conduct for the study of rare diseases; and although the benefits and harms of an intervention may be well recognized, its extent or significance is not always well assessed.12
Alternatives to randomized controlled clinical trials
In practice randomized controlled clinical trials often pose logistical challenges and ethical problems, which limit sample sizes.14,15 Therefore, alternatives to randomized controlled clinical trials are needed.
Patient preference trials
When patients or surgeons have strong treatment preferences and refuse randomization, patients may be willing to participate in a preference trial. A preference trial is a trial in which the patient is offered the treatment options rather than random assignment. Patients who have a clear preference are given their preferred treatment while patients without a preference are randomized.16–18 Thus both randomized and nonrandomized patients are studied together under the same protocol other than the treatment assignment.
There are a range of opinions as to the validity and usefulness of this study design. Some researchers believe they complement, rather than replace, randomized controlled clinical trials.17 Preference trials have been criticized for results that may not be generalizable due to the inherent bias in allowing patients with a strong preference to select their own treatment. Patients who prefer a specific treatment may be more compliant than others who have no such preference. This, and other potential impacts of a patient’s preference on actual outcome, is not yet a well-understood bias that can be accounted for. In addition, particularly in small studies, there is the inability to control fully for potential confounders that may differ significantly between treatment groups.17 Proponents of preference trials believe that subjects who choose their treatment may be more representative of patients in actual clinical practice and that they can provide insights into the patient decision-making process.16
Large multicenter trials
When an insufficient number of patients are enrolled in a randomized controlled clinical trial, researchers may choose to use large multicenter trials to increase the sample size.16 A multicenter controlled clinical trial carries the same requirements as a single-center trial and each site must follow the same protocol, with identical inclusion and exclusion criteria, randomization strategies, interventions, and methods for collecting and evaluating the outcomes.14 Nevertheless, some researchers have found variability in surgery and surgical techniques in multicenter trials.16
Observational studies
Given the difficulty of executing a randomized controlled trial, the next best level of evidence is produced by a good experimental study or well-designed cohort study, with the former having some, but not all, the features of a randomized controlled trial. Although there is widespread belief that observational studies are less valid, often overestimating the magnitude of treatment effects, there are at least several reports suggesting that good observational studies can provide the same level of internal validity as randomized trials.19,20
Cohort studies
Cohort studies evaluate a group or cohort at risk for disease. The evaluation may occur over time or at a single point in time. The latter is further described as a cross-sectional cohort study and is often used to collect data on the prevalence of disease. The second common use of cohort studies is to analyze the exposures that put subjects at risk for disease or interventions that reduce that risk. This requires an evaluation of the cohort at a minimum of two points in time. The study may be designed prospectively (Fig. 10.3) or retrospectively (Fig. 10.4). In the prospective cohort study, the investigator develops a hypothesis about variables that may impact the outcome under investigation, collects data about those risk factors, and then follows the cohort for development of the outcome of interest. For example, when the association between smoking and lung cancer was suspected, a prospective study design increased the strength of that causal association.
Case-control studies
Case-control studies (Fig. 10.5) differ from cohort studies in that two distinct groups of subjects are investigated. The first group (case) is selected by the presence of disease and the second (control) by its absence. In contrast, a single population at risk for disease is studied in a cohort design. Like cohort studies, the case-control design may be prospective or retrospective. The major strength of the case-control study is in the investigation of rare conditions or outcomes. Its weakness lies in the inability to assess incidence or prevalence of disease and its increased susceptibility to bias. Controls may be concurrent or historic, matched (on key variables such as age and sex) or unmatched.
Large-database analysis
Population-based research
Effectiveness versus efficacy
Clinicians need to have information on an intervention’s outcomes under real-world conditions, not just in the optimal setting.21,22 As we discussed in the prior section, case series and clinical trials performed across a few academic centers are at risk for selection bias, and the results represent outcomes under optimal conditions. National databases, on the other hand, include patients and physicians from all types of healthcare settings, allowing assessment of outcomes in the “real world.” This was demonstrated with the randomized clinical trials for carotid endarterectomy procedures (Fig. 10.6).22 The clinical trials reported a very low mortality rate for these procedures. However, after the clinical trials concluded, the mortality following carotid endarterectomy was substantially higher than that reported in the trials, even among those institutions that participated in the randomized studies. Mortality with carotid endarterectomy was also found to be inversely proportional to a center’s volume. Thus, clinical trials generally do not represent real-world surgical outcomes.22 An alternative, research using a national database, is a population-based approach that provides information on a procedure’s effectiveness under real-world conditions rather than just its efficacy in ideal situations.
The importance of power
Adequate sample size is another key component to outcomes research. True differences in outcomes can only be detected when research studies are adequately powered through a large-enough sample size.23,24 Plastic surgery faces the challenge of being a relatively low-volume group of providers compared to other specialties, such as those treating cardiovascular disease. Outcomes studies with small patient samples are often underpowered and at risk for a type II, or B, error. This statistical error happens when a study does not find a statistically significant difference between treatment groups when a difference truly exists.25 Chung et al. found more than 80% of “negative studies” in the Journal of Hand Surgery had powers of less than 0.80 to detect a 25% difference between treatment groups.26 There is a greater than 20% risk of missing a true difference between treatment groups when a study has less than 0.80 power. The primary reason for the low power among these studies was insufficient sample size.26 Large-database analyses can provide sufficient sample size to avoid an underpowered study.
Table 10.3 illustrates the importance of an adequate sample size in outcomes research. In this theoretical example, imagine a large group of physicians having performed a cosmetic procedure on 48 500 patients with 1500 complications (3% complication rate). Imagine a small group of plastic surgeons having performed the same procedure on 46 patients, and 4 had a complication (8% complication rate). This is not a statistically significant difference due to the small number of procedures performed by the plastic surgeons. Imagine, in a similar example, the complication rate for the large group of physicians remains at 3% (1500 patients with a complication) and, due to a slight change in sampling, the plastic surgeons had no complications on 46 patients (0% complication rate). This is also not a statistically significant difference because the study was underpowered in regard to the number of patients treated by plastic surgeons. In fact, the study sample could increase to 100 patients for the plastic surgeons with no complications and still there would not have been a statistically significant difference between provider groups.
Data sources
Clinical registries
Large databases in healthcare can be generally described as either a clinical registry or administrative claims data (Table 10.4). Clinical registries are designed for the purpose of collecting clinical data and have the advantage of providing detailed patient and treatment information. Many national clinical registries are disease-specific, such as Surveillance Epidemiology and End Results (SEER) registry and the National Comprehensive Cancer Network (NCCN) that monitor outcomes in cancer patients. Plastic surgery has a national clinical registry called Tracking Operations and Outcomes for Plastic Surgeons (TOPS), which is supported by the American Society of Plastic Surgeons and the American Board of Plastic Surgery. The primary purpose of TOPS is to facilitate monitoring the quality of surgical care in plastic surgery. These types of clinical registries can be invaluable to researchers who are interested in studying patient populations that may not be captured in claims data, such as younger patients not captured in Medicare or the self-pay cosmetic patients. Researchers should be aware of the potential limitations of data sources. For example, the SEER registries only capture information within the first 4 months of initial treatment, and the TOPS database relies on voluntary physician-reported data. However, a recent study supports the validity of the TOPS database.27
Table 10.4 Examples of clinical registries and administrative claims data
Data source | Description |
---|---|
Clinical registries | |
Surveillance, Epidemiology and End Results (SEER) registry | Sponsored by the National Cancer Institute and collects data on cancer incidence and survival for approximately 26% of the US population146 |
National Comprehensive Cancer Network (NCCN) | Alliance of the leading cancer centers in the US that are dedicated to improving the quality of cancer care147 |
National Surgical Quality Improvement Program (NSQIP) | Program is sponsored by the American College of Surgeons and includes academic and private-sector hospitals which desire to participate. Data include inpatient and outpatient visits among patients undergoing major surgical procedures148 |
Tracking Operations and Outcomes for Plastic Surgeons (TOPS) | Program is sponsored by the American Society of Plastic Surgeons, the Plastic Surgery Educational Foundation, and the American Board of Plastic Surgery. Board-certified plastic surgeons may participate. Inpatient and outpatient data are collected149 |
Administrative claims data | |
State–federal partnerships | State healthcare organizations submit datasets to the Agency for Healthcare and Research Quality150 |
State Inpatient Database | |
Nationwide Inpatient Sample | |
Kids Inpatient Database | |
State Ambulatory Surgery Database | |
Medicare151 | |
Part A | Inpatient hospital discharge abstracts |
Part B | Outpatient claims data |
Veterans Affairs (VA) data | |
Patient Treatment File (PTF) | Inpatient hospital discharge abstracts |
Outpatient Care Files (OCF) | Outpatient visits at VA-based clinics |
CosmetAssure152 | Insurance company that covers complications related to cosmetic surgery |
Administrative claims data
It is not possible to use claims data to evaluate cosmetic procedures since these procedures are self-pay. Researchers have used information from CosmetAssure to evaluate cosmetic surgery outcomes.27 CosmetAssure is an insurance policy sold nationally that covers medical and surgical complications from cosmetic surgery. There is a financial incentive to report a covered complication. However, clinical outcomes collected in the database are limited.
How to use large databases for research
Small-area variation
Prior to the 1970s, the rate of surgery for a population could not be determined, and data were limited to the number of cases performed at a given institution or by a specific surgeon. In 1973, Wennberg and Gittelsohn published a method for estimating the population served by a medical center, which allowed for the first time the calculation of procedure rates (e.g., the number of tonsillectomies per 100 000 people served).28,29 We now know that certain surgical procedures demonstrate wide and unexplained variations in regional and national rates (e.g., hysterectomy, prostatectomy, cesarean section) whereas other procedures are performed at similar rates (e.g., appendectomy, cholecystectomy). In general, those procedures whose risks and benefits are well established exhibit the least variability. Procedures with high variability suggest that the optimum management has yet to be established or adopted. Although small-area variations allow us to make observations about differences in procedure rates, they do not help explain these variations or tell us which rate is right. What it does suggest is that a more definitive evaluation needs to be undertaken to identify the optimal treatment strategy.30 The Dartmouth Atlas of Health Care is one of the best examples of how to use administrative data to examine variations in the rates of surgery in the US using data from the national Medicare database, provider files, and American Hospital Association.31
Volume–outcome analysis
Another field of investigation that falls under the broad heading of large-database analysis is the area of volume–outcome studies. There is growing evidence to suggest that surgical outcomes are better at high-volume institutions.32 The reason may in part be due to the skill of the surgeon but also reflects the hospital and systems that support a given population of patients. A landmark paper by Birkmeyer et al.33 described a significant correlation between surgeon volume and operative mortality in the US using Medicare data. Another example is the work by Roohan et al.34 on the 5-year survival of patients with breast cancer treated at low-, moderate-, and high-volume centers (Table 10.5). This work shows that there is as much as a 30% difference in the 5-year survival between low- and high-volume hospitals.
Table 10.5 Volume–outcome association for 5-year survival after breast cancer treatment
Hospital volume | Breast cancer cases/year | 5-year survival risk ratio |
---|---|---|
Very low | 1–10 | 1.60 |
Low | 11–50 | 1.30 |
Moderate | 51–150 | 1.19 |
High | >150 | 1.00 |
(Adapted from Roohan PJ, Bickell NA, Baptiste MS, et al: Hospital volume differences and five-year survival from breast cancer. Am J Public Health 1998;88:454–457.)
Epidemiology
Large databases are also useful tools to evaluate trends over time in surgical utilization and outcomes for a population. For example, the Nationwide Inpatient Sample was used to document a dramatic increase in the use of bariatric surgery in the US.35 The national SEER database has been used to assess sociodemographic differences in receipt of postmastectomy breast reconstruction36 and assess trends in the use of breast reconstruction after the Women’s Health and Cancer Rights Act.37 These studies found that the overall use of breast reconstruction across the US is low, that race/ethnicity is a significant predictor of receipt of postmastectomy reconstruction, and that the use of reconstruction did not increase significantly after the passing of the federal law that mandated insurance coverage of these procedures.
Examples of large-database analyses in plastic surgery
Many large national and state databases have had limited applications for plastic surgeons.38,39 The most obvious reason is that many of the procedures performed by plastic surgeons are not captured in these databases. For example, the Medicare database captures only patients older than 65 years who use Medicare as the primary payment mechanism.
Two authors have made an effort to use these databases to garner information on small-area variations useful to plastic surgeons. Gittelsohn and Powe used data from the state of Maryland to compare rates of elective surgery, such as septoplasty, rhinoplasty, reduction mammoplasty, and blepharoplasty.40 Significant variations were noted in rates of surgery. Explanatory variables were explored, and the authors concluded that income and race were significant predictors of surgery. Keller et al. have looked at variations in the rate of surgery for carpal tunnel syndrome in the state of Maine.41 They were able to identify a 3.5-fold difference in rates, and the authors concluded that the major driving factor is physician decision-making. We do not know the “right” rate of carpal tunnel surgery, but the data provided by studies such as this will enable us to begin to ask the appropriate questions.
Large databases have provided the source for cohort investigations on the relationship between breast reduction and a reduced risk for development of breast cancer. Boice et al.42 identified breast reduction patients in Sweden using hospital discharge data and linked with Swedish registries for cancer, death, and emigration and compared results with expected breast cancer incidence in the general population using the Swedish Nationwide Cancer Registry. The investigators found that women 7.5 years after reduction mammaplasty were at a statistically significant 28% decreased risk of cancer. The NCCN database has been used to evaluate the association between postmastectomy breast reconstruction and the delivery of adjuvant chemotherapy for breast cancer patients.43 The authors found that immediate-postmastectomy breast reconstruction does not appear to lead to omission of chemotherapy, but it is associated with a modest, but statistically significant, delay in initiating treatment. For most, it is unlikely that this modest delay has any clinical significance. Regarding cosmetic procedures, the TOPS and CosmetAssure databases have been used to assess outcomes with abdominoplasty and breast augmentation procedures.27 The low complication rates found in this study support the safety of these procedures when performed by plastic surgeons. However, the authors stress that surgeons should be aware of the overall higher complication rates associated with combining breast augmentation with other procedures (Fig. 10.7). In summary, these represent just a few examples of the potential use of large databases in plastic surgery research.