Questions to ask while reading a paper
What type of study is it?
Does the study population match the patients to whom the results of the study will be applied? Who was excluded from the study?
Is the hypothesis clearly stated? Does the study really test that hypothesis?
Can the predictor, outcome, and potential confounders be reliably measured? Are there limitations based on the data source?
Are there missing data? How does the study address this problem?
Could the results be explained by chance, bias, or confounding?
Are the study’s conclusions supported by the results?
Administrative Data
Administrative data is usually derived from claims data, or information collected in the process of billing for surgical care. Clinical episodes generally produce a bill for professional services (CPT codes), as well as bill for hospital or facility fees (ICD-9 codes). This billing information, or claims data, can be compiled into different data sources, for comparative analyses.
There are several strengths to using administrative data. It is readily available, and available in de-identified aggregate data sets, so there are few institutional review board (IRB) issues. Administrative data is compiled from several diverse sites, and different populations, resulting in large sample sizes and powerful analyses. These data sets span many years, so longitudinal analyses can be performed. As it is derived from billing data, economic data with regard to charges and cost may be included as well.
However, administrative data also has many weaknesses. There are significant issues with regard to the accuracy of the data. The data is limited by the codes available and how specific coding principles are applied. The researcher has no choice over the variables, and clinically relevant information you might want to obtain might not be coded for or it may be coded in a way that you would not want it to be coded. Administrative data is compiled by coders who are trying to maximize revenue, and this priority can affect the validity of the findings. There is concern for missing data when events that occur but are not billed for are not captured. Also, coding cannot always differentiate between a preoperative risk factor and a postoperative event, which limits the ability to do risk-adjusted analysis of postoperative complications.
Several examples of administrative data are listed, as well as their specific strengths and weaknesses:
UHC (University HealthSystem Consortium Data)
The University Health Consortium compiles hospital billing data from the 119 academic medical centers and their affiliated hospitals that are involved in this consortium. Cost data is included as well. Benchmarked data is available to participating hospitals to measure performance and for research purposes. The strengths of this data are the large amount of longitudinal data, the ease with which it can be analyzed, the Web-based reports that are readily available, and the relative homogeneity of the hospitals in the set. The major weaknesses are that not only is it administrative data and so has those inherent limitations described previously, but the generalizability of the findings is limited since the data only includes large academic medical centers.
NIS (National Inpatient Sample)
NIS is an administrative database that is part of the Healthcare Cost and Utilization Project (HCUP). This is an all-payer database of inpatient care in the United States and is publicly available for a minimal cost. It includes a 20% stratified sample of US community hospitals, including data from 45 states or 96 % of the US population. The sampling and weighting strategies can be taken into account to create national estimates. Data elements that could directly or indirectly identify individuals are excluded. Details are available at www.hcup-us.ahrq.gov/nisoverview.
Medicare Claims Data
Medicare claims data includes data on all Medicare patients and includes outpatient, inpatient, skilled nursing facilities, hospice, home health agencies, and everything else that Medicare pays for including Part D (prescription drugs). Medicare covers patients who are 65 years or older and a citizen or permanent resident of the United States. If you are not yet 65, you might also qualify for coverage if you have a disability or have end-stage renal disease.
Patient-identified data sets, limited data sets, and de-identified data sets are available. Using this data is quite complex and requires significant training and support from ResDAC (the Research Data Assistance Center) to access and use it. It is an enormously powerful data set given the extent of data captured in all settings of care and can be linked to other public data sets. Generalizability is limited as it only applies to patients with Medicare.
All-Payer Claims Data Sets
Certain states or regions have all-payer data sets available for patients receiving care in those areas. HCUP-SID (Healthcare Cost and Utilization Project State Inpatient Databases) are available for certain states, and these include all administrative data on all inpatient discharges in that state or region. Data sets are priced per state per year of data and range from $35 to $3,000. Companies like Premier, Inc., have aggregated all-payer data sets for 400+ hospitals and include drug information, as well as cost data or charge data. Companies like Healthgrades aggregate the statewide (HCUP-SID) and national (e.g., Centers for Medicare & Medicaid Services) data sets and then use proprietary methodology to rank hospitals.
Large Cohort Studies
SOS (Swedish Obese Subjects)
A cohort of 4,047 obese patients in Sweden was enrolled in this study from 1987 to 2001: 2,010 of the patients chose to undergo bariatric surgery and 2,037 patients were contemporaneously matched with the bariatric surgery group based on 18 matching variables (i.e., nonrandomized matched prospective controls). Important information continues to be learned from this observational cohort study as it continues over time, which is up past 20 years (with a lost to follow-up rate of only 36.2 % at 15 years.) However, the case mix, with 68 % vertical-banded gastroplasty, only 13 % gastric bypass (mostly open), and 19 % gastric banding (most with old bands and without the pars flaccida technique), does not reflect the types of procedures performed today.
LABS (Longitudinal Assessment of Bariatric Surgery)
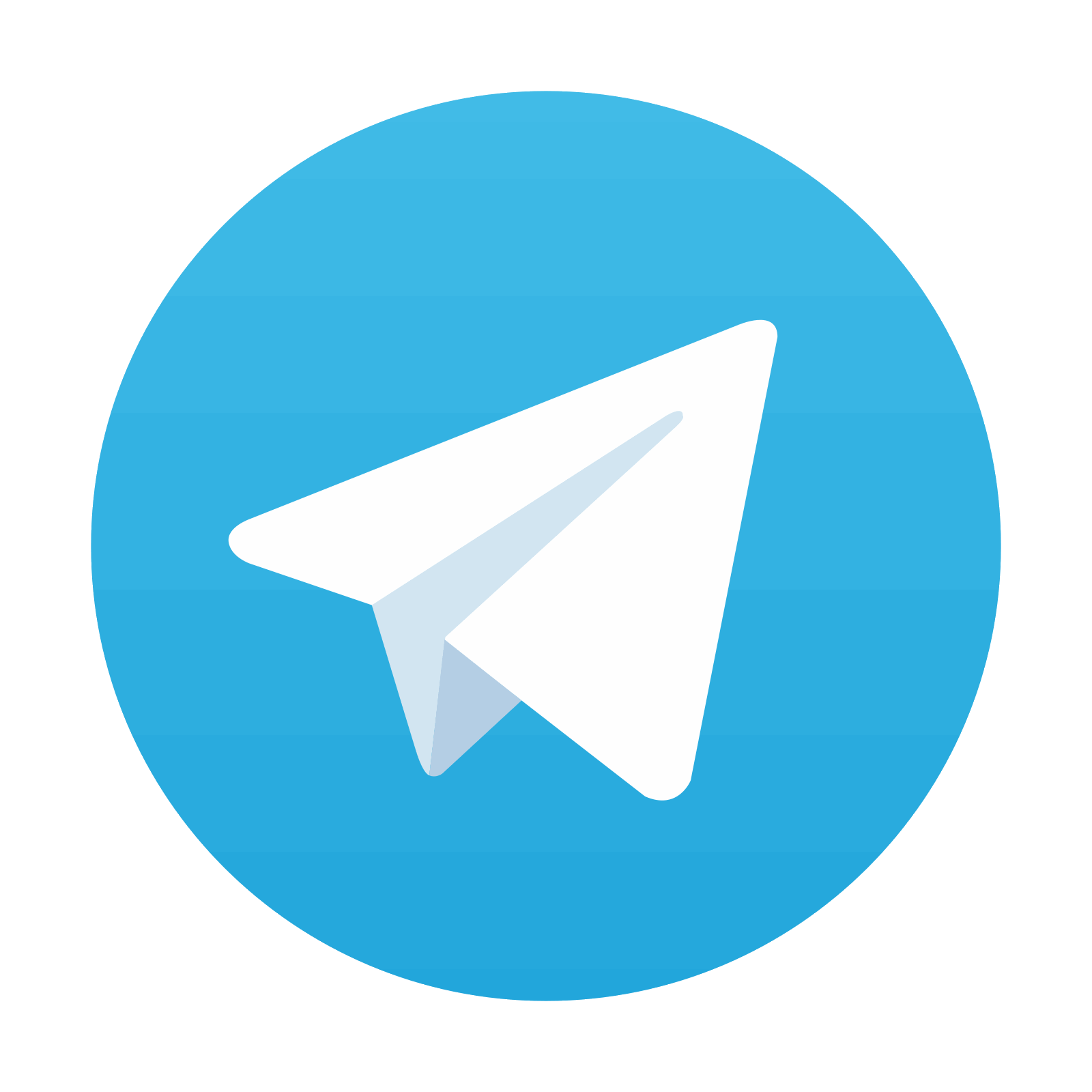
Stay updated, free articles. Join our Telegram channel

Full access? Get Clinical Tree
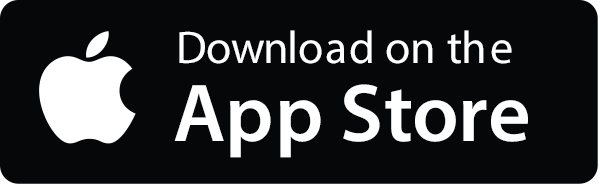
