This article explores the current options for the quantitative assessment of hypertrophic burn scars. It also introduces a novel type of randomized, controlled trial, which relies on heterogeneity of the subject population to improve the predictive value of personalized treatment strategies.
Key points
- •
Accurately measuring burn scar characteristics can aid clinical decision making but is critical for conducting meaningful research.
- •
Quantitative burn scar measurements include assessment of surface area, vascularity, pigmentation, thickness, and pliability.
- •
Randomized clinical trials to determine efficacy of therapy for hypertrophic scars are difficult to perform in burn patients but are critical to developing evidence-based guidelines and algorithms for treatment.
Introduction
Hypertrophic burn scars are considered common after burn injuries, but the true incidence is unknown. Reconstruction of hypertrophic burn scars remains challenging. Having a thorough understanding of the basics of quantitative burn scar research will allow physicians and scientists to more accurately treat and perform research on hypertrophic burn scars. Patients may respond differently to treatment of their scars.
Over the last decade there has been a rapidly growing interest in personalized medicine, and tailoring care based on patient characteristics and prior response to treatment has led to the development of the adaptive treatment strategy (ATS). ATS are predetermined decisions that select the next therapy based on current patient data in order to customize patient care and achieve better outcomes. ATS continue to show a great deal of promise in modifying patient care based on individual responses to treatment.
A relatively new method of researching ATS is the development of sequential multiple assignment randomized trials (SMARTs). At the core of this research, several groups of subjects are assigned to different initial treatments and, based on the response, continue initial treatment versus nonresponders who have additional medications, therapies, or procedures added to the regimen. This method of research yields data that inform the investigator about factors that play into response to treatment, what treatment has the most efficacy, and in what sequence the treatments obtain optimal outcome. The purpose of this article is to familiarize surgeons with SMARTs using subject-specific and audience-specific examples with the intent of increasing use in clinical research.
Introduction
Hypertrophic burn scars are considered common after burn injuries, but the true incidence is unknown. Reconstruction of hypertrophic burn scars remains challenging. Having a thorough understanding of the basics of quantitative burn scar research will allow physicians and scientists to more accurately treat and perform research on hypertrophic burn scars. Patients may respond differently to treatment of their scars.
Over the last decade there has been a rapidly growing interest in personalized medicine, and tailoring care based on patient characteristics and prior response to treatment has led to the development of the adaptive treatment strategy (ATS). ATS are predetermined decisions that select the next therapy based on current patient data in order to customize patient care and achieve better outcomes. ATS continue to show a great deal of promise in modifying patient care based on individual responses to treatment.
A relatively new method of researching ATS is the development of sequential multiple assignment randomized trials (SMARTs). At the core of this research, several groups of subjects are assigned to different initial treatments and, based on the response, continue initial treatment versus nonresponders who have additional medications, therapies, or procedures added to the regimen. This method of research yields data that inform the investigator about factors that play into response to treatment, what treatment has the most efficacy, and in what sequence the treatments obtain optimal outcome. The purpose of this article is to familiarize surgeons with SMARTs using subject-specific and audience-specific examples with the intent of increasing use in clinical research.
Basics of quantitative burn scar research
The true incidence of hypertrophic scarring after burns is unknown but is relatively common. Dealing with long-term sequelae of burns and trying to mitigate functional deficits and cosmetic deformities can be challenging. The literature on this topic is generally full of case reports, techniques articles, and innovations, but there is a lack of consistency of the interstudy research methodology. The goal here is to familiarize the reader with a basic understanding of the quantitative research methods in burn reconstruction in hopes of a more succinct use of mainstream techniques for conducting research. This is not meant as a comprehensive overview but rather an introduction. A more in-depth discussion, including clinical scar assessment and the varied scar scales available to researchers can be found.
Instrumentation for Objective Burn Scar Measurement
Quantitative burn scar research consists of measuring a combination of 4 key components: color, surface area, height or depth, and pliability.
The researcher has a choice of several objective color measurement devices, including the Chroma Meter (Minolta, Osaka, Japan), the Spectrometer (Cortex technology, Hadsund, Denmark), and the Mexameter (MX18, Courage & Khazaka Electronic GmbH, Cologne, Germany). The Chroma Meter has been used most often at our institution and is frequently used in published literature, and has been validated in several trials ; therefore a brief description is in order. The Chroma Meter contains a xenon lamp that emits a white beam, which is picked up by photodetectors after interacting with the tissue. It gives information about the brightness, red-green, and blue-yellow spectrums of the tissue. The Chroma Meter correlates with the Vancouver Scar Scale vascularity index and is useful in bridging the clinical or objective scar measurement divide.
Surface area is typically captured by a simple length-times-width calculation of square centimeters of involved tissue. This becomes problematic in scars with significant height or depth and surface irregularities that obscure accurate measurement. Although this methodology will still suffice for most studies, it is worth mentioning 3-dimensional imaging because it will likely play a significant role in the future of scar research. Devices such as Vectra (Canfield Scientific, Fairfield, NJ, USA), Crisalix (Parc Scientifique, Lausanne, Switzerland), and the Vivid 900 3-D digitizer (Konica Minolta, Milton Keynes, UK) have various volume or surface area measuring capabilities and can show before or after treatment changes in scar volume. They are somewhat cost-prohibitive and used for aesthetic surgery or research but will likely make a debut into hypertrophic burn scars in the near future.
Height or depth parameters involve volume calculations that, as previously mentioned, have limitations of needing a starting point (usually the lowest depression in scar or adjacent normal skin); however, they do not have the ability to measure the depth of the scar beneath the skin. Ultrasound technology has been at the forefront of burn scar research, and the DermaScan-C (Cortex Technologies, Hadsund, Denmark) is the most commonly used in the literature. These high frequency (20 MHz) probes have the ability to distinguish between hypertrophic scars, normal scars, and unaffected skin based on subtle differences in architecture or depth.
Finally, pliability remains of significant interest in scar research because this characteristic can mean the difference between an unsightly and a functionally limiting scar, particularly near joints. The Cutometer (MPA580; Courage + Khazada, Cologne, Germany) functions by applying a probe to the scar, which exerts 500 mbar of negative pressure in 3 bursts. This measures the viscoelastic properties of the skin and has been shown to have good inter-rater or intra-rater reliability and validity on hypertrophic scars.
Overall, these 4 quantitative methods provide the basis for objective burn scar measurement and should be in the forethought of researchers starting a new research project, clinical trial, or a quality improvement project in a burn scar population. Technology is ever changing, and newer methodologies for quantitative burn scar measurement will continue to evolve. It is important as a research community to stay vigilant in reporting standards to ensure comparability across studies and to afford these patients the best care possible as knowledge of treatment strategies continues to mature.
The sequential multiple assignment randomized trial option
Background
Personalized medicine has recently become a hot topic, particularly with recent advances in genomics, proteomics, and pharmacology. Intuitively, health care providers know that some patients respond differently to treatment than others. These idiosyncrasies are multifactorial with genetic components that, for the most part, have yet to be elucidated. However, it still behooves the clinician to try and pick the best treatment plan for the patient despite the risk of nonresponse, partial response, or even harm. Finding the right medication, therapy, or procedure for a given patient based on their history, current medical condition, and prior response to treatment is at the core of personalized medicine. Traditionally, randomized controlled trials (RCTs) will test a new intervention versus standard of care or placebo using 2 to 3 cohorts. SMARTs are able to start with a similar goal of testing multiple treatment arms but have the novel ability to start with several groups and rerandomize through the study, measuring which combinations of treatments are best for each subject ( Fig. 1 ). Over the last 20 years, several papers have been published using SMART research methodology to try and find the best treatments, or combinations thereof, notably in psychiatry and cancer research. For the sake this discussion and its intended audience, the treatment of hypertrophic burn scars is used as an illustration. However, this could easily be applied to other research topics within reconstructive or cosmetic plastic surgery, such as facial rejuvenation, use of artificial skin, timing of burn excision, and timing and technique of cleft lip repair, as well as the broader surgical discipline, including critical care sedation and pharmacotherapy, chronic wound care, Barrett esophagus management, skin cancer treatment, transplant, traumatic brain injury, and so forth.
Lavori and colleagues first brought the concepts of ATS, randomization, and flexible treatment strategies together. This and other papers described the new methodology in psychiatry. Indeed, chronic medical conditions tend to lend themselves to SMART methodology more than acute conditions because the latter frequently do not require multiple treatment strategies. For surgical procedures in which the offending pathologic entity can be effectively removed without the need for chronic management or multiple treatments, these descriptions will rarely apply. When designed properly, this methodology can also directly compare multiple therapeutic agents versus standard therapy rather than 1 agent versus a placebo. SMARTs can also help answer questions such as does success of first treatment predict or affect success of secondary treatment and does the type of first treatment change overall response to the various second treatments?
Designing a Sequential Multiple Assignment Randomized Trial
With the underlying purpose of developing ATS, SMARTs generate large amounts of prospective data for interpretation. When trying to find the best treatment or series of treatments, comparing the different arms of the study will generate questions such as does group A have better outcomes than group B or does group F have a shorter length of stay than group C? Due to the complex design and resources required for implementation of this type of research methodology, experts recommend beginning with a pilot study. This will identify key issues and allow troubleshooting of the design before starting a full-scale SMART. The SMART trial differs from typical RCT in that RTCs test a hypothesis, whereas SMART trials generate a hypothesis. The pilot study should be thought of as a beta test rather than a smaller version of the trial. Data gathered from the pilot should not be used to answer the research questions but rather to identify problems with the methodology and create solutions. Typical problems with patient compliance, staff understanding of research protocols, and data gathering should be addressed before beginning a full-scale SMART. If they are not addressed beforehand, this can lead to data gaps and failure of certain treatment arms to yield meaningful information.
After identifying the topic of research and making treatment arms, the next step is addressing the primary tailoring variable (PTV). Essentially, the PTV is how to select the next treatment. This is usually dichotomous, highly customizable, and can be objective (scar pliability, C-reactive protein level) or subjective (subject reports fewer symptoms of itching or pain). The research team needs to set parameters regarding how to assess these subjects, how to quantify variables, and the timing of reassessment, as well as the possibility of changing treatment of early nonresponders. PTVs need to be customized to fit the individual study; however, fundamental discussions must occur no matter what the area of study. Of particular interest is what is known about the topic in published literature that can be used to identify if and when the subject has responded to treatment. Is the gold standard of measurement something that the facility can accommodate? Will the subject receive treatment until they have responded, or is a measurable nonresponse or partial response also an indication to proceed to the next treatment?
Randomization
SMARTs can be randomized early with each subject assigned to a sequence of events at the beginning of the trial (static), or with evaluation after each treatment dictating the next form of intervention (dynamic). In a mental health scenario, the subject who responds well to an antidepressant may be scheduled to be switched to cognitive behavioral therapy (CBT) or be scheduled to have CBT added to the treatment (static model). In comparison, if the subject responds to the antidepressant, they may be kept in this treatment arm or changed to CBT due to partial or nonresponse (dynamic model). They may also show some improvement with the antidepressant and be considered to have maxed out the benefits, so additional treatments could be added. The type of randomization selected by the research team should take into account consequences of changing therapy from the subject perspective. Will discontinuing the antidepressant result in subjects having more symptoms and being unwilling to continue in the trial?
Example of Sequential Multiple Assignment Randomized Trial Design
Consider a hypothetical scenario using the static model in which researchers are studying the effect of laser therapy on subjects with hypertrophic scars as a result of burn injury. For the sake of demonstration, each subject has a single area of concern that will be addressed over the course of the trial. Pulse dye laser (PDL), fractional ablative carbon dioxide laser (CO2), and medical management (MM), that is, compressive garments and topical emollients, will be the 3 treatments allotted for 3 months each. Fig. 1 demonstrates all of the available treatment arms for subjects in this scenario, and compares this with an RCT.
Before entering the study, subjects will complete quality-of-life surveys and questionnaires to quantify how their scars cause functional and cosmetic deformity, and to elicit symptoms of pruritus, pain, and paresthesia. Objective measurements include scar thickness, elasticity, and color. These questionnaires and measurements will be repeated before starting the next arm of the trial (every 3 months), and will be used to follow patients into post-treatment convalescence. To answer the question of whether the sequence of treatments changes the outcome, the following study arms are assigned:
Arm 1: MM-PDL-CO2
Arm 2: MM-CO2-PDL
Arm 3: PDL-MM-CO2
Arm 4: PDL-CO2-MM
Arm 5: CO2-MM-PDL
Arm 6: CO2-PDL-MM.
To answer the question of whether 1 laser has more therapeutic benefit compared with another, multiple treatment arms will involve use of just 1 laser with 2 treatments:
Arm 7: CO2-CO2-MM
Arm 8: MM-CO2-CO2
Arm 9: CO2-MM-CO2
Arm 10: PDL-PDL-MM
Arm 11: MM-PDL-PDL
Arm 12: PDL-MM-PDL.
It should be noted that there are multiple additional options for treatment arms that are not listed here that are seen in Fig. 1 . The options include arms that are nothing but 1 type of treatment (CO2-CO2-CO2), and arms that have 2 treatments consisting of MM. There are 2 reasons these options for treatment are not present in this example:
- 1.
The workplace is a common locale for patients to suffer burn injuries. Many of these patients are eager to return to work, or have workers compensation cases that require the quickest treatment, making prolonged time spent in MM treatment unfeasible.
- 2.
As standard of care continues to evolve, it is important to ensure that clinical equipoise is maintained. If patients not in the study would have received 2 or more treatments with laser therapy within the course of a year, then patient enrolled in the study should receive at least this to maintain standard of care. They should not get undertreated simply because they are enrolled in a clinical trial.
Potential Difficulties
How does the researcher deal with the subject who wishes to change therapy in the middle of the trial? Before beginning a pilot study, it is important to have informed consent performed by the Principal Investigator or qualified representative who can detail all aspects of the research and discuss with each subject the importance of the trial, and so prevent as much noncompliance and dropout as possible. The pilot study should identify key issues that subjects raise so that dropout rates do not significantly affect the results. As with any research, there will be a certain amount of dropout for various reasons. However statistical methods exist for dealing with missing data at the end of a SMART.
Dropout rates of certain arms and refusal to continue treatment can provide additional information, particularly if the cause can be related to nonresponse, or difficulty with logistics related to treatment. In the first scenario, subjects may not return to clinic at the appropriate time because the treatment is not working. If a subject did not respond to PDL treatment, why would the patient return for an additional PDL treatment followed by an MM assignment? This dropout or these missing data can be considered a nonresponse, and the subject could be progressed to another arm of the trial. Dropout can also clue researchers in to the larger problem of logistics. If subjects are not returning to clinic because of scheduling conflicts, lack of reminders, and so forth, it is worth investigating during the pilot study to try and prevent this occurring during a full-scale SMART. Keeping subjects in treatment arms for prolonged periods of time may accommodate scheduling issues.
Data Analysis and Calculating Enrollment for the Pilot Study
SMART data are complex to analyze due to the large and diverse amount of data produced; the large number of treatment sequences; the dynamic nature of time, which changes subject variables over subsequent treatment stages; and, most problematically, the need to identify an optimal treatment sequence for each subject, not simply compare fixed treatments between all subjects.
The usual and traditional ways to analyze clinical trial data are ill suited to handle the complexities of SMART data. Hence, novel methodology has been developed to cope, bringing together techniques and ideas from diverse areas and fields, such as computer science and casual estimation. The methods may be classified into roughly 3 categories :
- 1.
Regression methods are based on the familiar and well-understood regression techniques while allowing the implementation of state-of-the-art machine-learning regression techniques. However, rather than estimating the final outcome for every 1 of the possible treatment sequences, these often focus on estimating each subject’s best possible outcome, or at least which treatment should be given at each stage to obtain the best outcome. These methods also work iteratively, proceeding backwards from the final outcome, to estimate what each subject’s best treatment would have been at each stage to gain the optimal outcome. The iterative method avoids (at least somewhat) the problem of attempting to estimate the outcomes of a possibly very large number of possible treatment sequences because only the much smaller number of treatments allowed at a particular stage are the main consideration at each regression corresponding to that stage.
- 2.
Value maximization methods attempt to estimate, for a given adaptive treatment rule, the subject’s outcome under that treatment rule. Then, this predicted outcome is maximized over treatment rules in some permitted set to find the optimal rule (within the permitted set). These methods may also proceed iteratively backwards, breaking down the process into steps corresponding to each treatment stage.
- 3.
Planning methods use models to simulate subject trajectories under different postulated rules. The optimum rule can then be investigated. These methods significantly depend on good theoretic models of the processes involved at each treatment stage and are, therefore, perhaps less relevant for the analysis of clinical study data.
Each category of method and, indeed, each technique within each category has its own peculiar strengths and weaknesses. Hence, the selected analysis technique should ultimately depend intricately on the particular trial and aims.
The treatment rule obtained from a SMART may be regarded as a black-box in which the clinician may input an individual’s variables and be given the optimal treatment sequentially as the treatment process unfolds. Alternatively, if the clinician is uncomfortable with the interpretability of such a black-box rule, the treatment rule may be chosen to be optimal among a set of rules that the clinician believes have a good medical interpretation; for example, a rule defined using a small number of dichotomous variables (age<40 years, male gender, body mass index>30). Such rules may be more insightful for scientific knowledge such as the mechanism of the disease and treatment.
Unfortunately, inference and sample size calculations are somewhat difficult for SMARTs. Many of the problematic issues arise due to so-called nonregularity of the estimators. That is to say, small changes in a subject’s variables at some point could result in an entirely treatment sequence being embarked on. This lack of smoothness, such as small changes that might have disproportionately drastic results, makes standard statistical analysis extremely difficult. This is an area of active statistical research and exciting progress is being made.
This progress notwithstanding, given current methodology, it is perhaps best to simply regard these trials as hypothesis-generating, both for the personalized dynamic treatment rule obtained and any interesting hypotheses that can be concluded from examining this rule (eg, all individuals>35 years with burn scars benefit most from CO2 laser treatment 3 months after injury). Then, a classical RCT may be performed to confirm these hypotheses.
Although there are, as yet, no straightforward sample size calculations, interesting progress is also being made to address this gap, with some formulas being proposed for specific circumstances. Additionally, simulations show that SMART trials give a plethora of information from relatively small samples (eg, fewer than 150 individuals, with 2 decision points and 2 treatments at each decision point). Methods to calculate how many individuals should be sampled in a certain SMART, other than simulation, might be the perusal and analysis (with SMART analytical machinery) of observational data or conducting an appropriate pilot study. Another method could be to power the trial to answer a classic question. For the burn trial example, one could sample enough individuals to answer, using standard statistical tests, the question of whether the MM-MM-MM significantly differs from the other permitted treatments that involve some combination of CO2 and PDL. This way, the trial is likely to be a success, at least with regard to the classic question, with the added bonus of many additional conclusions obtained from the SMART analyses.
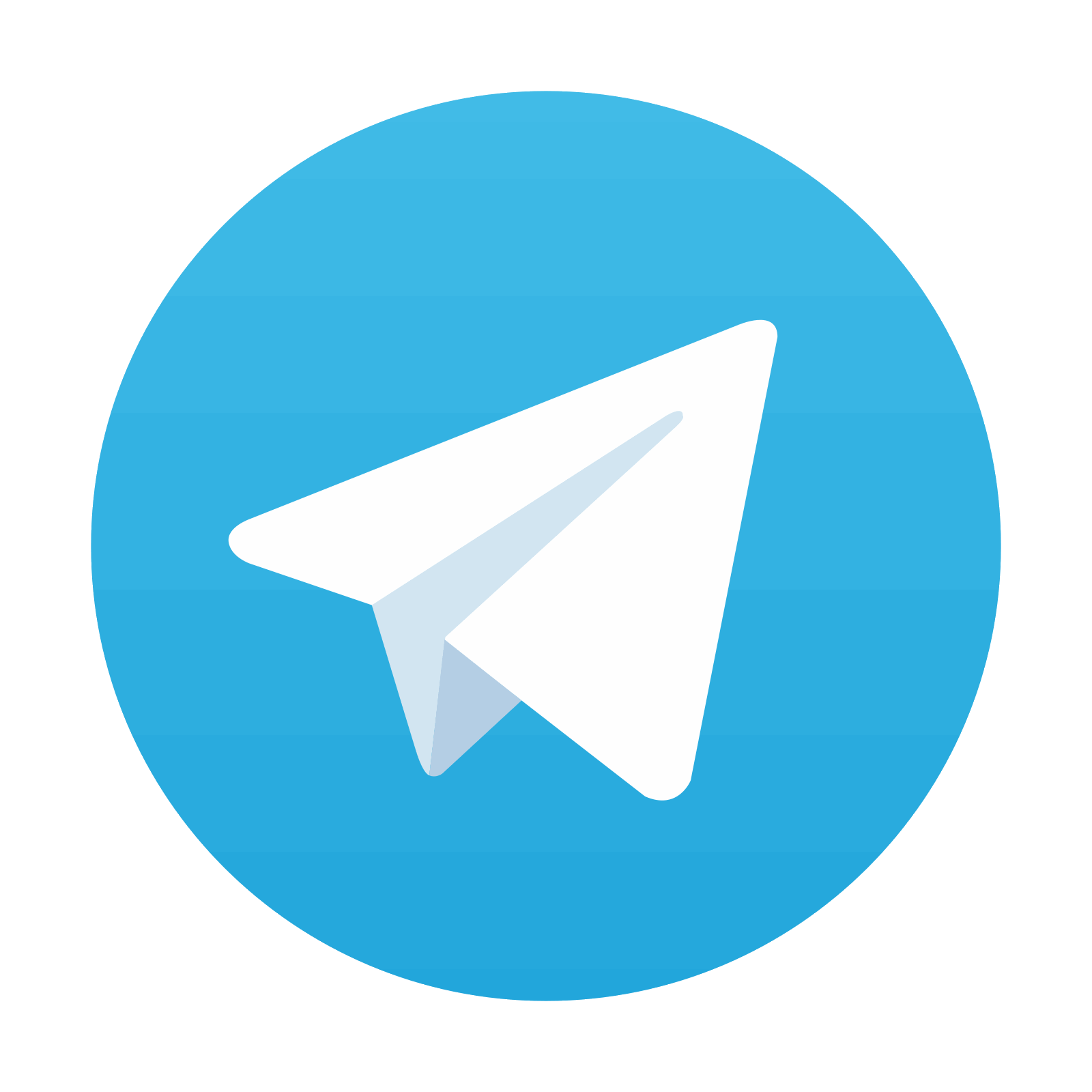
Stay updated, free articles. Join our Telegram channel

Full access? Get Clinical Tree
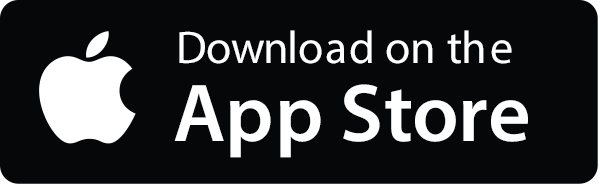
