© Springer Science+Business Media New York 2015
Gerald Brandacher (ed.)The Science of Reconstructive TransplantationStem Cell Biology and Regenerative Medicine10.1007/978-1-4939-2071-6_1010. Bayesian Classifier and Molecular Marker Platforms for Immune Monitoring
(1)
Walter Reed National Military Medical Center, Bethesda, MD, USA
(2)
Uniformed Services University of Health Sciences, 4301 Jones Bridge Road, Bethesda, MD, USA
(3)
George Washington University, Washington, DC, USA
(4)
Naval Medical Research Center, Silver Spring, MD, USA
Keywords
BayesianClassifierMachine learningTransplantationBiomarkerImmune statusMolecular markerAbbreviations
BBNs
Bayesian belief networks
CDS
Clinical decision support
USRDS
(United States Renal Data System) database
OPO
Organ Procurement Organization
TG
Transplant glomerulopathy
PPV
Positive predictive value
WRTC, www.wrtc.org
Washington Regional Transplant Community
PSAs
Public service announcements
AUC
Under the curve
VCAs
Vascularized composite allografts
Introduction
Solid organ transplantation is the definitive treatment for end-stage organ failure, and vascularized composite allografts (VCAs) are an increasingly important restorative option. However, clinical decisions regarding allocation or postoperative treatment can be difficult to make. This has stimulated research investigating applications designed to analyze large amounts of data, show how that data affects outcomes, and assist in the decision-making process. As has been done in cardiology and oncology, our research uses machine learning techniques to produce a clinically relevant prognostic tool [1–3].
Machine learning uses logical algorithms that allow computers to recognize complex patterns within datasets. These patterns can then be represented mathematically into clinical decision support (CDS) tools. In the area of transplantation, machine learning has been used to estimate the likelihood of delayed graft function, transplant glomerulopathy (TG), and allograft survival.
Many types of statistical techniques can yield CDS tools, including neural networks, nomograms, and tree modeling [4–7]. Nomograms tend to use multivariate analysis in their formation, but can be limited by the number of variables they may incorporate. Neural networks and tree modeling use machine learning, but variables are selected based on univariate analysis. While both techniques identify variables significantly associated with outcome, each may leave out variables that are not predictive by themselves but can yield prognostic information when considered in combination with additional variables. In addition, both neural networks and tree modeling require the input data to be complete, and as such, are intolerant of missing data common in the clinical setting. Bayesian belief networks (BBNs), on the other hand, are designed to accommodate missing information by generating an array of conditional interdependencies. In this fashion, a joint probability distribution function can be generated which allows all variables (or features) to be displayed graphically within the same model.
Bayesian analysis has been used to examine kidney transplant outcomes with standard criteria, extended criteria, and donation after cardiac death. BBNs have been used in medicine for over a decade and are ideal for analysis of datasets containing missing information and rare events, such as elucidating disease processes, selecting ideal alternative therapies, and estimating the likelihood of certain outcomes [8] including 90-day outcomes following liver transplantation [9].
This chapter reviews their use as applied to the diagnosis of TG using biomarkers , estimating the likelihood of renal allograft survival when using deceased donor grafts, and developing a better understanding of how beliefs within ethnic populations influence organ donation within those communities. Additionally, we describe the future expanded role of machine learning in determining immune status across transplantation.
Bayesian Modeling Applied to Molecular Markers
There is an extensive area of research surrounding biomarkers and their application in wound healing, oncology, and transplantation [10–12] . In oncology, particularly for breast cancer, biomarkers such as human epidermal growth factor receptor 2 (HER2) and multigene assays are already being used to personalize therapy [13, 14]. There is also considerable interest in developing biomarkers for the early detection of breast cancer before it is evident on mammography [11].
In transplantation, biomarkers have been studied to diagnose TG. TG is a disease of the kidney allograft that, despite having a low prevalence (< 10 %), has a high morbidity with approximately 50 % of grafts that develop TG failing within 10 years [15, 16]. While the etiology has been researched extensively, no causative factor has been found for which there is specific treatment aside from increasing immunosuppression. As such, Elster et al. [12] used a BBN approach to develop a learning model which compares gene transcripts from both the immune and fibrosis pathways in patients who developed TG and those who had stable function as defined by 6 months posttransplant with no change in renal function and no significant histological or clinical abnormalities. Their model confirmed several mechanisms which are already known, but also uncovered novel relationships such as the interdependence of ICAM-1, IL-10, CCL-3, and the development of TG, as well as the association between C4d grade and increased expression of VCAM-1, MMP-9, MMP-7, and LAMC2 in TG development. Furthermore, the models developed for gene panel 1(immune pathway) and gene panel 2 (fibrosis) were able to estimate the probability of developing TG based on available evidence. When internal cross validations of the models were performed, the predictive accuracy as measured by area under the curve (AUC) was 0.875 for GP1 and 0.859 for GP2. While these models and biomarker profiles do not fully elucidate the mechanisms involved in TG development, as in oncology, they offer the potential to direct therapy specific to a patient’s particular pathology .
Bayesian Models as Applied to Kidney Transplantation
Population-based research is common in transplantation, as is the use of machine learning to develop predictive models for renal allograft function and allocation. The tools currently used to make final allocation decisions are inadequate and subjective, which can result in suboptimal graft survival . As more extended criteria are available from donors and donation following cardiac graft failure, it becomes increasingly important to develop relevant CDS tools designed to identify those donor/recipient graft pairings that are likely to fail. Machine learning has enabled the development of a prognostic model that incorporates multiple variables for a systems approach to organ allocation and made the process more objective.
This study used a BBN to create a prognostic model for deceased donor renal allograft transplantation. This probabilistic approach is useful when dealing with large databases, can tolerate missing information, and can graphically describe the probability distributions of outcomes based on the conditional interdependence of known information [17]. In other words, this type of statistical analysis allows for the use of an unlimited number of variables and identifies not only the relationship between each variable and the targeted outcome but also the relative contribution and inter-variable relationships to the probability of each outcome [9].
To create this model, first time renal transplant patients over the age of 18 and only receiving a kidney were selected from the United States Renal Data System (USRDS) database [18
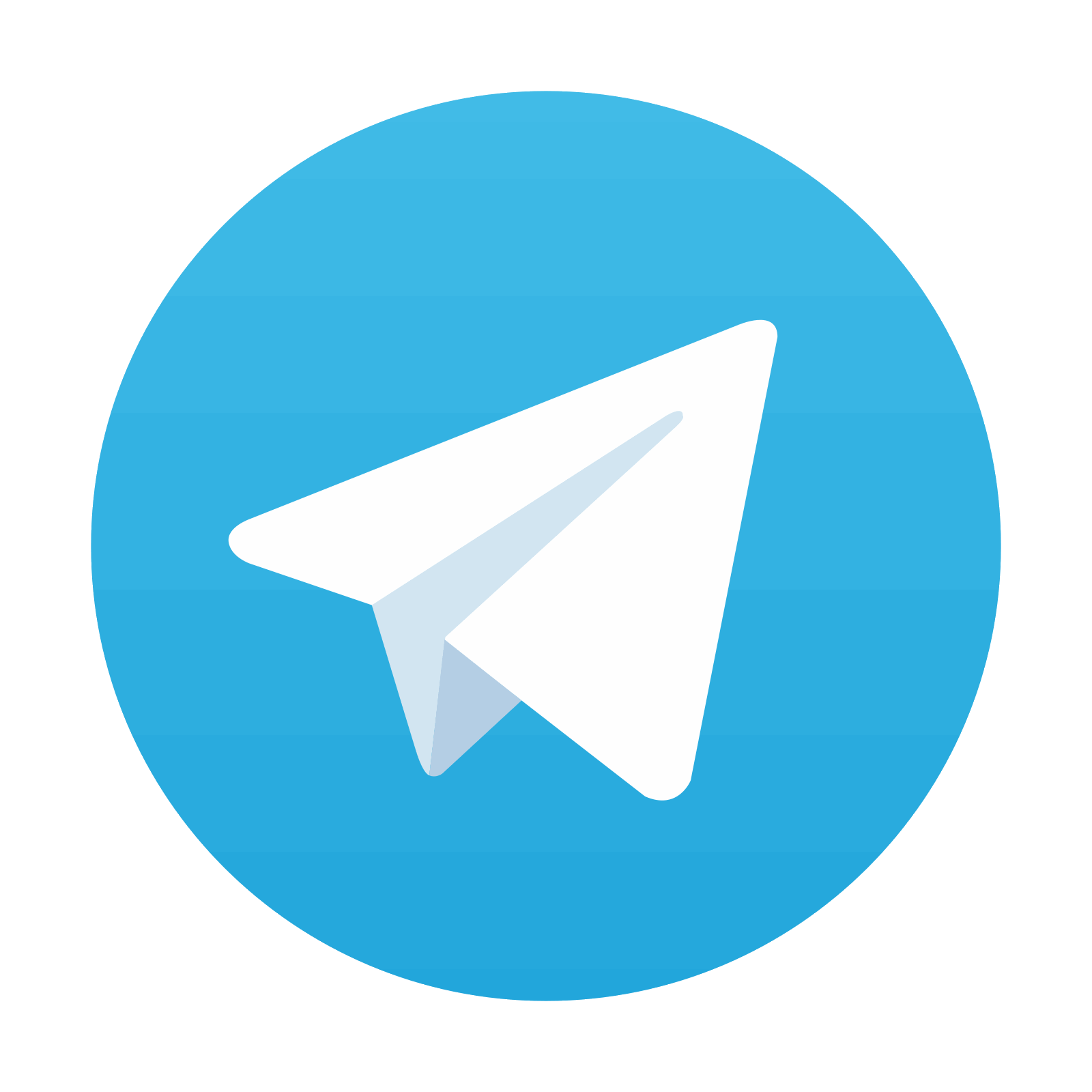
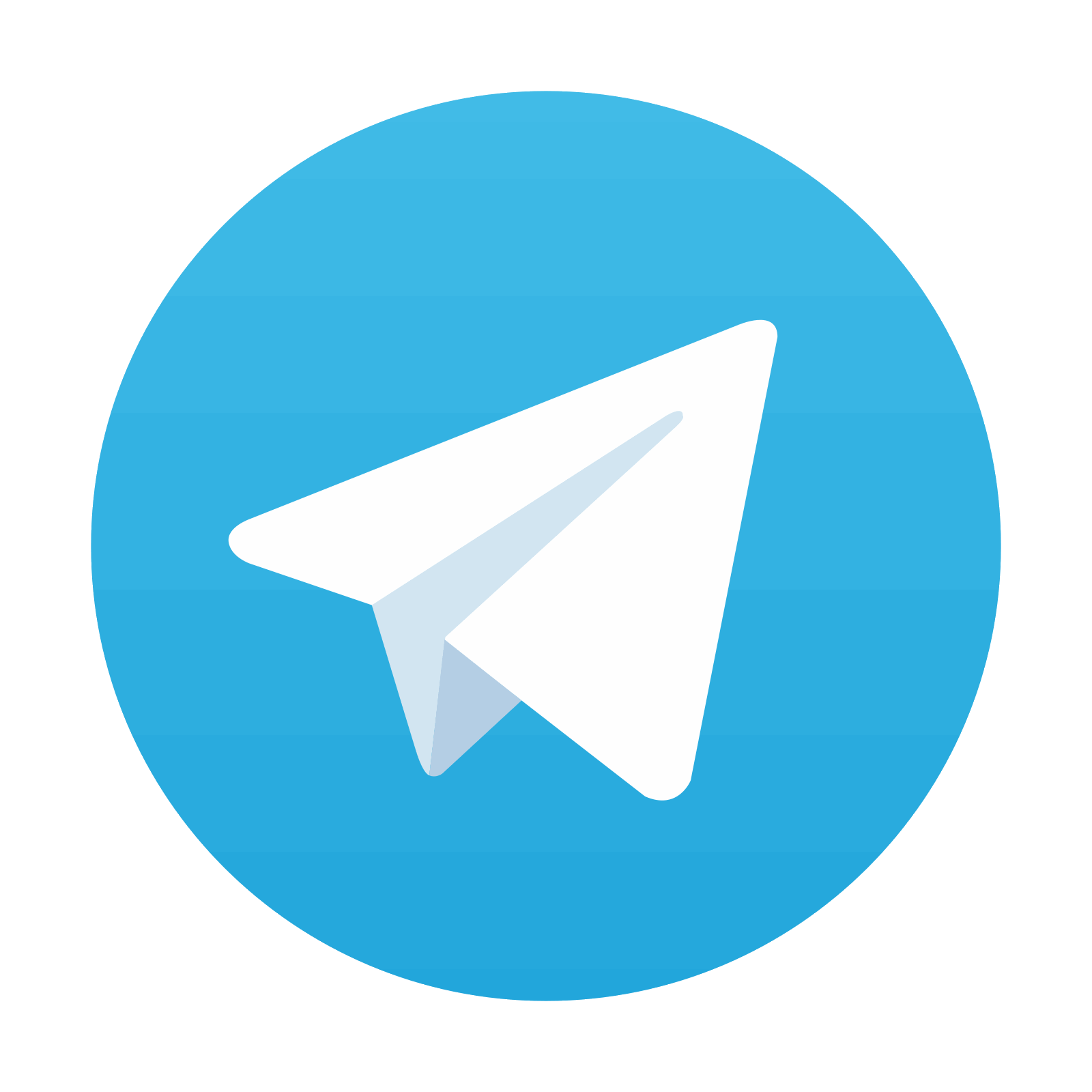
Stay updated, free articles. Join our Telegram channel

Full access? Get Clinical Tree
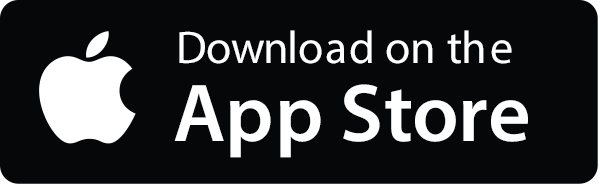
